Open Access
ARTICLE
AutoRhythmAI: A Hybrid Machine and Deep Learning Approach for Automated Diagnosis of Arrhythmias
Department of Computer Science and Engineering, SRM Institute of Science and Technology—Vadapalani Campus, Chennai, Tamil Nadu, India
* Corresponding Author: S. Jayanthi. Email:
Computers, Materials & Continua 2024, 78(2), 2137-2158. https://doi.org/10.32604/cmc.2024.045975
Received 13 September 2023; Accepted 27 October 2023; Issue published 27 February 2024
Abstract
In healthcare, the persistent challenge of arrhythmias, a leading cause of global mortality, has sparked extensive research into the automation of detection using machine learning (ML) algorithms. However, traditional ML and AutoML approaches have revealed their limitations, notably regarding feature generalization and automation efficiency. This glaring research gap has motivated the development of AutoRhythmAI, an innovative solution that integrates both machine and deep learning to revolutionize the diagnosis of arrhythmias. Our approach encompasses two distinct pipelines tailored for binary-class and multi-class arrhythmia detection, effectively bridging the gap between data preprocessing and model selection. To validate our system, we have rigorously tested AutoRhythmAI using a multimodal dataset, surpassing the accuracy achieved using a single dataset and underscoring the robustness of our methodology. In the first pipeline, we employ signal filtering and ML algorithms for preprocessing, followed by data balancing and split for training. The second pipeline is dedicated to feature extraction and classification, utilizing deep learning models. Notably, we introduce the ‘RRI-convoluted transformer model’ as a novel addition for binary-class arrhythmias. An ensemble-based approach then amalgamates all models, considering their respective weights, resulting in an optimal model pipeline. In our study, the VGGRes Model achieved impressive results in multi-class arrhythmia detection, with an accuracy of 97.39% and firm performance in precision (82.13%), recall (31.91%), and F1-score (82.61%). In the binary-class task, the proposed model achieved an outstanding accuracy of 96.60%. These results highlight the effectiveness of our approach in improving arrhythmia detection, with notably high accuracy and well-balanced performance metrics.Keywords
Cite This Article
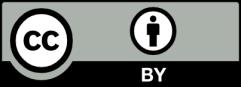