Open Access
ARTICLE
Enhancing Image Description Generation through Deep Reinforcement Learning: Fusing Multiple Visual Features and Reward Mechanisms
School of Cyber Security, Gansu University of Political Science and Law, Lanzhou, 730070, China
* Corresponding Author: Qiyuan Wang. Email:
(This article belongs to the Special Issue: Machine Vision Detection and Intelligent Recognition)
Computers, Materials & Continua 2024, 78(2), 2469-2489. https://doi.org/10.32604/cmc.2024.047822
Received 19 November 2023; Accepted 11 January 2024; Issue published 27 February 2024
Abstract
Image description task is the intersection of computer vision and natural language processing, and it has important prospects, including helping computers understand images and obtaining information for the visually impaired. This study presents an innovative approach employing deep reinforcement learning to enhance the accuracy of natural language descriptions of images. Our method focuses on refining the reward function in deep reinforcement learning, facilitating the generation of precise descriptions by aligning visual and textual features more closely. Our approach comprises three key architectures. Firstly, it utilizes Residual Network 101 (ResNet-101) and Faster Region-based Convolutional Neural Network (Faster R-CNN) to extract average and local image features, respectively, followed by the implementation of a dual attention mechanism for intricate feature fusion. Secondly, the Transformer model is engaged to derive contextual semantic features from textual data. Finally, the generation of descriptive text is executed through a two-layer long short-term memory network (LSTM), directed by the value and reward functions. Compared with the image description method that relies on deep learning, the score of Bilingual Evaluation Understudy (BLEU-1) is 0.762, which is 1.6% higher, and the score of BLEU-4 is 0.299. Consensus-based Image Description Evaluation (CIDEr) scored 0.998, Recall-Oriented Understudy for Gisting Evaluation (ROUGE) scored 0.552, the latter improved by 0.36%. These results not only attest to the viability of our approach but also highlight its superiority in the realm of image description. Future research can explore the integration of our method with other artificial intelligence (AI) domains, such as emotional AI, to create more nuanced and context-aware systems.Keywords
Cite This Article
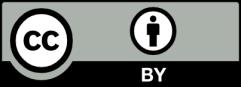