Open Access
ARTICLE
The Influence of Air Pollution Concentrations on Solar Irradiance Forecasting Using CNN-LSTM-mRMR Feature Extraction
Department of Computer Engineering, Istanbul University-Cerrahpasa, Istanbul, 34300, Turkey
* Corresponding Author: Ramiz Gorkem Birdal. Email:
Computers, Materials & Continua 2024, 78(3), 4015-4028. https://doi.org/10.32604/cmc.2024.048324
Received 04 December 2023; Accepted 29 January 2024; Issue published 26 March 2024
Abstract
Maintaining a steady power supply requires accurate forecasting of solar irradiance, since clean energy resources do not provide steady power. The existing forecasting studies have examined the limited effects of weather conditions on solar radiation such as temperature and precipitation utilizing convolutional neural network (CNN), but no comprehensive study has been conducted on concentrations of air pollutants along with weather conditions. This paper proposes a hybrid approach based on deep learning, expanding the feature set by adding new air pollution concentrations, and ranking these features to select and reduce their size to improve efficiency. In order to improve the accuracy of feature selection, a maximum-dependency and minimum-redundancy (mRMR) criterion is applied to the constructed feature space to identify and rank the features. The combination of air pollution data with weather conditions data has enabled the prediction of solar irradiance with a higher accuracy. An evaluation of the proposed approach is conducted in Istanbul over 12 months for 43791 discrete times, with the main purpose of analyzing air data, including particular matter (PM10 and PM25), carbon monoxide (CO), nitric oxide (NOX), nitrogen dioxide (NO₂), ozone (O₃), sulfur dioxide (SO₂) using a CNN, a long short-term memory network (LSTM), and MRMR feature extraction. Compared with the benchmark models with root mean square error (RMSE) results of 76.2, 60.3, 41.3, 32.4, there is a significant improvement with the RMSE result of 5.536. This hybrid model presented here offers high prediction accuracy, a wider feature set, and a novel approach based on air concentrations combined with weather conditions for solar irradiance prediction.Keywords
Cite This Article
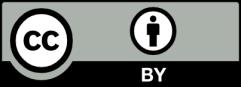