Open Access
ARTICLE
Intelligent Design of High Strength and High Conductivity Copper Alloys Using Machine Learning Assisted by Genetic Algorithm
1 Metallurgical & Materials Engineering Department, Indian Institute of Technology, Kharagpur, West Bengal, 721302, India
2 Computer Science & Engineering Department, Indian Institute of Technology, Kharagpur, West Bengal, 721302, India
3 Vice Chancellor Office, Birla Institute of Technology (BIT) Mesra, Ranchi, Jharkhand, 835215, India
* Corresponding Author: Indranil Manna. Email:
(This article belongs to the Special Issue: Application of Soft Computing in Techniques in Materials Development)
Computers, Materials & Continua 2024, 79(1), 1727-1755. https://doi.org/10.32604/cmc.2024.042752
Received 10 June 2023; Accepted 28 October 2023; Issue published 25 April 2024
Abstract
Metallic alloys for a given application are usually designed to achieve the desired properties by devising experiments based on experience, thermodynamic and kinetic principles, and various modeling and simulation exercises. However, the influence of process parameters and material properties is often non-linear and non-colligative. In recent years, machine learning (ML) has emerged as a promising tool to deal with the complex interrelation between composition, properties, and process parameters to facilitate accelerated discovery and development of new alloys and functionalities. In this study, we adopt an ML-based approach, coupled with genetic algorithm (GA) principles, to design novel copper alloys for achieving seemingly contradictory targets of high strength and high electrical conductivity. Initially, we establish a correlation between the alloy composition (binary to multi-component) and the target properties, namely, electrical conductivity and mechanical strength. Catboost, an ML model coupled with GA, was used for this task. The accuracy of the model was above 93.5%. Next, for obtaining the optimized compositions the outputs from the initial model were refined by combining the concepts of data augmentation and Pareto front. Finally, the ultimate objective of predicting the target composition that would deliver the desired range of properties was achieved by developing an advanced ML model through data segregation and data augmentation. To examine the reliability of this model, results were rigorously compared and verified using several independent data reported in the literature. This comparison substantiates that the results predicted by our model regarding the variation of conductivity and evolution of microstructure and mechanical properties with composition are in good agreement with the reports published in the literature.Graphic Abstract
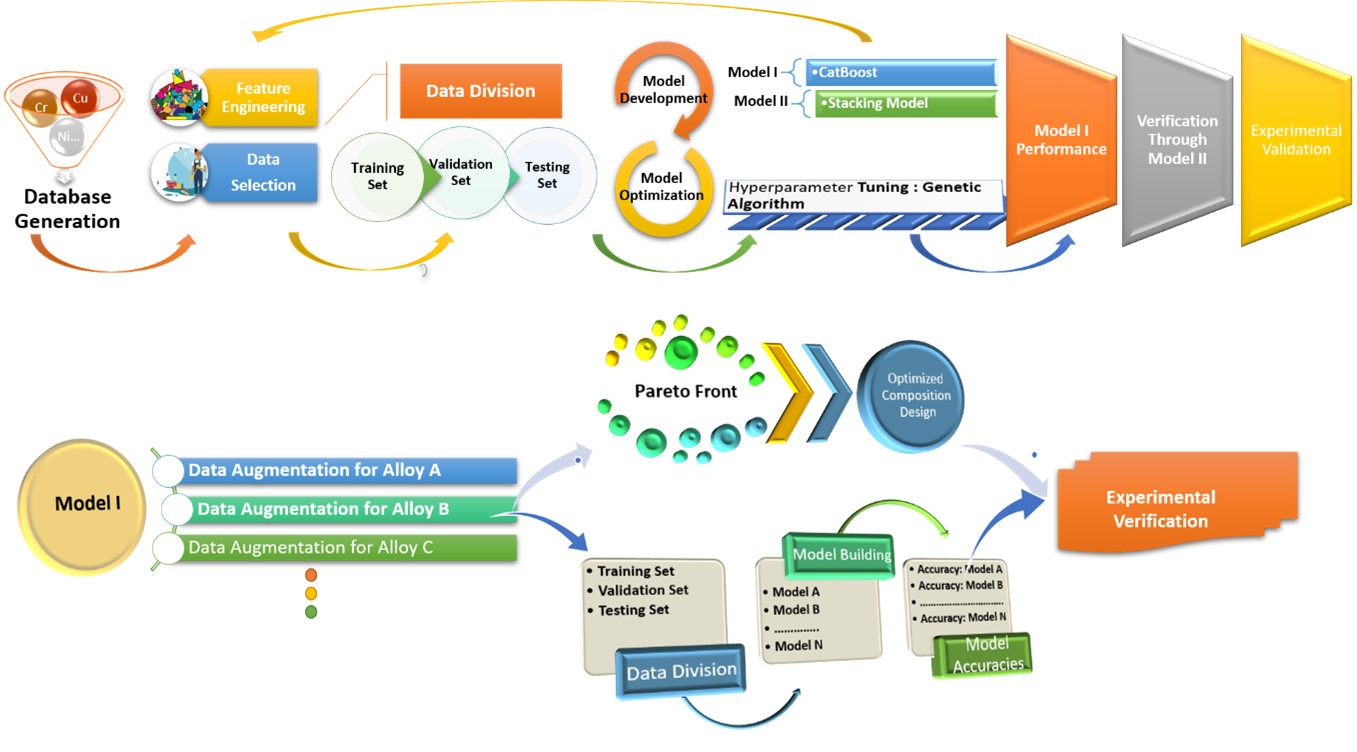
Keywords
Supplementary Material
Supplementary Material FileCite This Article
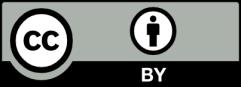