Open Access
ARTICLE
An Innovative K-Anonymity Privacy-Preserving Algorithm to Improve Data Availability in the Context of Big Data
1 State Key Laboratory of Public Big Data, College of Computer Science and Technology, Guizhou University, Guiyang, 550025, China
2 College of Information Engineering, Guizhou Open University, Guiyang, 550025, China
3 Guizhou Academy of Tobacco Science, Guiyang, 550025, China
* Corresponding Author: Yuling Chen. Email:
(This article belongs to the Special Issue: Security and Privacy for Blockchain-empowered Internet of Things)
Computers, Materials & Continua 2024, 79(1), 1561-1579. https://doi.org/10.32604/cmc.2023.046907
Received 18 October 2023; Accepted 12 December 2023; Issue published 25 April 2024
Abstract
The development of technologies such as big data and blockchain has brought convenience to life, but at the same time, privacy and security issues are becoming more and more prominent. The K-anonymity algorithm is an effective and low computational complexity privacy-preserving algorithm that can safeguard users’ privacy by anonymizing big data. However, the algorithm currently suffers from the problem of focusing only on improving user privacy while ignoring data availability. In addition, ignoring the impact of quasi-identified attributes on sensitive attributes causes the usability of the processed data on statistical analysis to be reduced. Based on this, we propose a new K-anonymity algorithm to solve the privacy security problem in the context of big data, while guaranteeing improved data usability. Specifically, we construct a new information loss function based on the information quantity theory. Considering that different quasi-identification attributes have different impacts on sensitive attributes, we set weights for each quasi-identification attribute when designing the information loss function. In addition, to reduce information loss, we improve K-anonymity in two ways. First, we make the loss of information smaller than in the original table while guaranteeing privacy based on common artificial intelligence algorithms, i.e., greedy algorithm and 2-means clustering algorithm. In addition, we improve the 2-means clustering algorithm by designing a mean-center method to select the initial center of mass. Meanwhile, we design the K-anonymity algorithm of this scheme based on the constructed information loss function, the improved 2-means clustering algorithm, and the greedy algorithm, which reduces the information loss. Finally, we experimentally demonstrate the effectiveness of the algorithm in improving the effect of 2-means clustering and reducing information loss.Keywords
Cite This Article
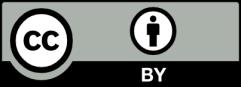