Open Access
ARTICLE
Spinal Vertebral Fracture Detection and Fracture Level Assessment Based on Deep Learning
1 The Electrical Engineering College, Guizhou University, Guiyang, 550025, China
2 Guizhou Provincial Orthopedics Hospital, Guiyang, 550025, China
* Corresponding Author: Yuhang Wang. Email:
Computers, Materials & Continua 2024, 79(1), 1377-1398. https://doi.org/10.32604/cmc.2024.047379
Received 03 November 2023; Accepted 13 March 2024; Issue published 25 April 2024
Abstract
This paper addresses the common orthopedic trauma of spinal vertebral fractures and aims to enhance doctors’ diagnostic efficiency. Therefore, a deep-learning-based automated diagnostic system with multi-label segmentation is proposed to recognize the condition of vertebral fractures. The whole spine Computed Tomography (CT) image is segmented into the fracture, normal, and background using U-Net, and the fracture degree of each vertebra is evaluated (Genant semi-qualitative evaluation). The main work of this paper includes: First, based on the spatial configuration network (SCN) structure, U-Net is used instead of the SCN feature extraction network. The attention mechanism and the residual connection between the convolutional layers are added in the local network (LN) stage. Multiple filtering is added in the global network (GN) stage, and each layer of the LN decoder feature map is filtered separately using dot product, and the filtered features are re-convolved to obtain the GN output heatmap. Second, a network model with improved SCN (M-SCN) helps automatically localize the center-of-mass position of each vertebra, and the voxels around each localized vertebra were clipped, eliminating a large amount of redundant information (e.g., background and other interfering vertebrae) and keeping the vertebrae to be segmented in the center of the image. Multilabel segmentation of the clipped portion was subsequently performed using U-Net. This paper uses VerSe’19, VerSe’20 (using only data containing vertebral fractures), and private data (provided by Guizhou Orthopedic Hospital) for model training and evaluation. Compared with the original SCN network, the M-SCN reduced the prediction error rate by 1.09% and demonstrated the effectiveness of the improvement in ablation experiments. In the vertebral segmentation experiment, the Dice Similarity Coefficient (DSC) index reached 93.50% and the Maximum Symmetry Surface Distance (MSSD) index was 4.962 mm, with accuracy and recall of 95.82% and 91.73%, respectively. Fractured vertebrae were also marked as red and normal vertebrae were marked as white in the experiment, and the semi-qualitative assessment results of Genant were provided, as well as the results of spinal localization visualization and 3D reconstructed views of the spine to analyze the actual predictive ability of the model. It provides a promising tool for vertebral fracture detection.Keywords
Cite This Article
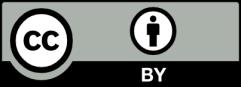