Open Access
ARTICLE
Ghost Module Based Residual Mixture of Self-Attention and Convolution for Online Signature Verification
1 Department of Computer Technology, School of Computer Science and Engineering, Shenyang Jianzhu University, Shenyang, 110168, China
2 Liaoning Province Big Data Management and Analysis Laboratory of Urban Construction, Shenyang Jianzhu University, Shenyang, 110168, China
3 Shenyang Branch of National Special Computer Engineering Technology Research Center, Shenyang Jianzhu University, Shenyang, 110168, China
* Corresponding Author: Shuai Yuan. Email:
Computers, Materials & Continua 2024, 79(1), 695-712. https://doi.org/10.32604/cmc.2024.048502
Received 09 December 2023; Accepted 26 February 2024; Issue published 25 April 2024
Abstract
Online Signature Verification (OSV), as a personal identification technology, is widely used in various industries. However, it faces challenges, such as incomplete feature extraction, low accuracy, and computational heaviness. To address these issues, we propose a novel approach for online signature verification, using a one-dimensional Ghost-ACmix Residual Network (1D-ACGRNet), which is a Ghost-ACmix Residual Network that combines convolution with a self-attention mechanism and performs improvement by using Ghost method. The Ghost-ACmix Residual structure is introduced to leverage both self-attention and convolution mechanisms for capturing global feature information and extracting local information, effectively complementing whole and local signature features and mitigating the problem of insufficient feature extraction. Then, the Ghost-based Convolution and Self-Attention (ACG) block is proposed to simplify the common parts between convolution and self-attention using the Ghost module and employ feature transformation to obtain intermediate features, thus reducing computational costs. Additionally, feature selection is performed using the random forest method, and the data is dimensionally reduced using Principal Component Analysis (PCA). Finally, tests are implemented on the MCYT-100 datasets and the SVC-2004 Task2 datasets, and the equal error rates (EERs) for small-sample training using five genuine and forged signatures are 3.07% and 4.17%, respectively. The EERs for training with ten genuine and forged signatures are 0.91% and 2.12% on the respective datasets. The experimental results illustrate that the proposed approach effectively enhances the accuracy of online signature verification.Keywords
Cite This Article
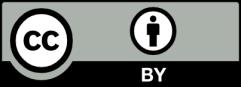