Open Access
ARTICLE
Automatic Road Tunnel Crack Inspection Based on Crack Area Sensing and Multiscale Semantic Segmentation
1 College of Mechanical and Electrical Engineering, Central South University, Changsha, 410083, China
2 Department of Geotechnical, Guangdong Hualu Transportation Technology Company Limited, Guangzhou, 510000, China
3 National Engineering Research Center of High-Speed Railway Construction Technology, Changsha, 410075, China
* Corresponding Author: Jilin He. Email:
Computers, Materials & Continua 2024, 79(1), 1679-1703. https://doi.org/10.32604/cmc.2024.049048
Received 26 December 2023; Accepted 06 March 2024; Issue published 25 April 2024
Abstract
The detection of crack defects on the walls of road tunnels is a crucial step in the process of ensuring travel safety and performing routine tunnel maintenance. The automatic and accurate detection of cracks on the surface of road tunnels is the key to improving the maintenance efficiency of road tunnels. Machine vision technology combined with a deep neural network model is an effective means to realize the localization and identification of crack defects on the surface of road tunnels. We propose a complete set of automatic inspection methods for identifying cracks on the walls of road tunnels as a solution to the problem of difficulty in identifying cracks during manual maintenance. First, a set of equipment applied to the real-time acquisition of high-definition images of walls in road tunnels is designed. Images of walls in road tunnels are acquired based on the designed equipment, where images containing crack defects are manually identified and selected. Subsequently, the training and validation sets used to construct the crack inspection model are obtained based on the acquired images, whereas the regions containing cracks and the pixels of the cracks are finely labeled. After that, a crack area sensing module is designed based on the proposed you only look once version 7 model combined with coordinate attention mechanism (CA-YOLO V7) network to locate the crack regions in the road tunnel surface images. Only subimages containing cracks are acquired and sent to the multiscale semantic segmentation module for extraction of the pixels to which the cracks belong based on the DeepLab V3+ network. The precision and recall of the crack region localization on the surface of a road tunnel based on our proposed method are 82.4% and 93.8%, respectively. Moreover, the mean intersection over union (MIoU) and pixel accuracy (PA) values for achieving pixel-level detection accuracy are 76.84% and 78.29%, respectively. The experimental results on the dataset show that our proposed two-stage detection method outperforms other state-of-the-art models in crack region localization and detection. Based on our proposed method, the images captured on the surface of a road tunnel can complete crack detection at a speed of ten frames/second, and the detection accuracy can reach 0.25 mm, which meets the requirements for maintenance of an actual project. The designed CA-YOLO V7 network enables precise localization of the area to which a crack belongs in images acquired under different environmental and lighting conditions in road tunnels. The improved DeepLab V3+ network based on lightweighting is able to extract crack morphology in a given region more quickly while maintaining segmentation accuracy. The established model combines defect localization and segmentation models for the first time, realizing pixel-level defect localization and extraction on the surface of road tunnels in complex environments, and is capable of determining the actual size of cracks based on the physical coordinate system after camera calibration. The trained model has high accuracy and can be extended and applied to embedded computing devices for the assessment and repair of damaged areas in different types of road tunnels.Keywords
Cite This Article
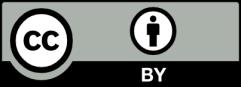