Open Access
ARTICLE
YOLO-MFD: Remote Sensing Image Object Detection with Multi-Scale Fusion Dynamic Head
School of Computer Science, Hunan University of Technology, Zhuzhou, 412007, China
* Corresponding Author: Wenqiu Zhu. Email:
(This article belongs to the Special Issue: Machine Vision Detection and Intelligent Recognition)
Computers, Materials & Continua 2024, 79(2), 2547-2563. https://doi.org/10.32604/cmc.2024.048755
Received 17 December 2023; Accepted 25 March 2024; Issue published 15 May 2024
Abstract
Remote sensing imagery, due to its high altitude, presents inherent challenges characterized by multiple scales, limited target areas, and intricate backgrounds. These inherent traits often lead to increased miss and false detection rates when applying object recognition algorithms tailored for remote sensing imagery. Additionally, these complexities contribute to inaccuracies in target localization and hinder precise target categorization. This paper addresses these challenges by proposing a solution: The YOLO-MFD model (YOLO-MFD: Remote Sensing Image Object Detection with Multi-scale Fusion Dynamic Head). Before presenting our method, we delve into the prevalent issues faced in remote sensing imagery analysis. Specifically, we emphasize the struggles of existing object recognition algorithms in comprehensively capturing critical image features amidst varying scales and complex backgrounds. To resolve these issues, we introduce a novel approach. First, we propose the implementation of a lightweight multi-scale module called CEF. This module significantly improves the model’s ability to comprehensively capture important image features by merging multi-scale feature information. It effectively addresses the issues of missed detection and mistaken alarms that are common in remote sensing imagery. Second, an additional layer of small target detection heads is added, and a residual link is established with the higher-level feature extraction module in the backbone section. This allows the model to incorporate shallower information, significantly improving the accuracy of target localization in remotely sensed images. Finally, a dynamic head attention mechanism is introduced. This allows the model to exhibit greater flexibility and accuracy in recognizing shapes and targets of different sizes. Consequently, the precision of object detection is significantly improved. The trial results show that the YOLO-MFD model shows improvements of 6.3%, 3.5%, and 2.5% over the original YOLOv8 model in Precision, map@0.5 and map@0.5:0.95, separately. These results illustrate the clear advantages of the method.Keywords
Cite This Article
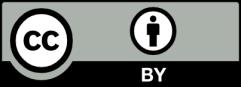