Open Access
ARTICLE
QoS Routing Optimization Based on Deep Reinforcement Learning in SDN
1 State Key Laboratory of Networking and Switching Technology, Beijing University of Posts and Telecommunications, Beijing, 100876, China
2 Marketing Service Center, State Grid Jiangsu Electric Power Co., Ltd., Nanjing, 220000, China
3 Customer Service Center, State Grid Co., Ltd., Nanjing, 211161, China
* Corresponding Author: Ao Xiong. Email:
Computers, Materials & Continua 2024, 79(2), 3007-3021. https://doi.org/10.32604/cmc.2024.051217
Received 29 February 2024; Accepted 09 April 2024; Issue published 15 May 2024
Abstract
To enhance the efficiency and expediency of issuing e-licenses within the power sector, we must confront the challenge of managing the surging demand for data traffic. Within this realm, the network imposes stringent Quality of Service (QoS) requirements, revealing the inadequacies of traditional routing allocation mechanisms in accommodating such extensive data flows. In response to the imperative of handling a substantial influx of data requests promptly and alleviating the constraints of existing technologies and network congestion, we present an architecture for QoS routing optimization with in Software Defined Network (SDN), leveraging deep reinforcement learning. This innovative approach entails the separation of SDN control and transmission functionalities, centralizing control over data forwarding while integrating deep reinforcement learning for informed routing decisions. By factoring in considerations such as delay, bandwidth, jitter rate, and packet loss rate, we design a reward function to guide the Deep Deterministic Policy Gradient (DDPG) algorithm in learning the optimal routing strategy to furnish superior QoS provision. In our empirical investigations, we juxtapose the performance of Deep Reinforcement Learning (DRL) against that of Shortest Path (SP) algorithms in terms of data packet transmission delay. The experimental simulation results show that our proposed algorithm has significant efficacy in reducing network delay and improving the overall transmission efficiency, which is superior to the traditional methods.Keywords
Cite This Article
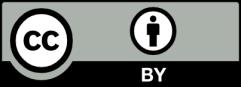