Open Access
ARTICLE
A New Industrial Intrusion Detection Method Based on CNN-BiLSTM
College of Computer Science and Technology, Shenyang University of Chemical Technology, Shenyang, 110142, China
* Corresponding Author: Qiang Fu. Email:
Computers, Materials & Continua 2024, 79(3), 4297-4318. https://doi.org/10.32604/cmc.2024.050223
Received 31 January 2024; Accepted 09 April 2024; Issue published 20 June 2024
Abstract
Nowadays, with the rapid development of industrial Internet technology, on the one hand, advanced industrial control systems (ICS) have improved industrial production efficiency. However, there are more and more cyber-attacks targeting industrial control systems. To ensure the security of industrial networks, intrusion detection systems have been widely used in industrial control systems, and deep neural networks have always been an effective method for identifying cyber attacks. Current intrusion detection methods still suffer from low accuracy and a high false alarm rate. Therefore, it is important to build a more efficient intrusion detection model. This paper proposes a hybrid deep learning intrusion detection method based on convolutional neural networks and bidirectional long short-term memory neural networks (CNN-BiLSTM). To address the issue of imbalanced data within the dataset and improve the model’s detection capabilities, the Synthetic Minority Over-sampling Technique-Edited Nearest Neighbors (SMOTE-ENN) algorithm is applied in the preprocessing phase. This algorithm is employed to generate synthetic instances for the minority class, simultaneously mitigating the impact of noise in the majority class. This approach aims to create a more equitable distribution of classes, thereby enhancing the model’s ability to effectively identify patterns in both minority and majority classes. In the experimental phase, the detection performance of the method is verified using two data sets. Experimental results show that the accuracy rate on the CICIDS-2017 data set reaches 97.7%. On the natural gas pipeline dataset collected by Lan Turnipseed from Mississippi State University in the United States, the accuracy rate also reaches 85.5%.Keywords
Cite This Article
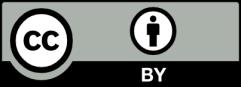