Open Access
ARTICLE
PPP: Prefix-Based Popularity Prediction for Efficient Content Caching in Contentcentric Networks
1 College of Computer Science and Technology, Henan Polytechnic University, Jiaozuo, China
2 College of Computer Science and Technology, Tianjin University, Tianjin, China
3 Investment Department II DT Capital, Beijing, China
Computer Systems Science and Engineering 2018, 33(4), 259-265. https://doi.org/10.32604/csse.2018.33.259
Abstract
In the Content-Centric Networking (CCN) architecture, popular content can be cached in some intermediate network devices while being delivered, and the following requests for the cached content can be efficiently handled by the caches. Thus, how to design in-network caching is important for reducing both the traffic load and the delivery delay. In this paper, we propose a caching framework of Prefix-based Popularity Prediction (PPP) for efficient caching in CCN. PPP assigns a lifetime (in a cache) to the prefix of a name (of each cached object) based on its access history (or popularity), which is represented as a Prefix-Tree (PT). We demonstrate PPP’s predictability of content popularity in CCN by both traces and simulations. The evaluation results show that PPP can achieve higher cache hits and less traffic load than traditional caching algorithms (i.e., LRU and LFU). Also, its performance gain increases with users of high mobility.Keywords
Cite This Article
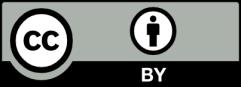