Open Access
ARTICLE
Prediction of College Students’ Physical Fitness Based on K-Means Clustering and SVR
Department of Physical Education, Hohai University, Nanjing 210098, China
* Corresponding Author: Email:
Computer Systems Science and Engineering 2020, 35(4), 237-246. https://doi.org/10.32604/csse.2020.35.237
Abstract
In today’s modern society, the physical fitness of college students is gradually declining. In this paper, a prediction model for college students’ physical fitness is established, in which support vector regression (SVR) and k-means clustering are combined together for the prediction of college students’ fitness. Firstly, the physical measurement data of college students are classified according to gender and class characteristics. Then, the k-means clustering method is used to classify the physical measurement data of college students. Next, the physical characteristics of college students are extracted by SVR to establish the prediction model of physical indicators, and the model for predicting college students’ fitness can be obtained after scoring their physical fitness levels. Finally, based on college physical test data of students at a university in China, the prediction results show that the method has high predictive accuracy compared to other methods.Keywords
Cite This Article
P. Tang, Y. Wang and N. Shen, "Prediction of college students’ physical fitness based on k-means clustering and svr," Computer Systems Science and Engineering, vol. 35, no.4, pp. 237–246, 2020. https://doi.org/10.32604/csse.2020.35.237Citations
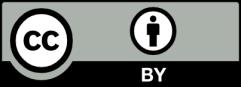