Open Access
ARTICLE
A Generative Adversarial Networks for Log Anomaly Detection
1 School of Computer Science, Wuhan University, Wuhan, 430072, China
2 Institute of Information Engineering, Chinese Academy of Sciences, Beijing, 100093, China
* Corresponding Author: Shi Ying. Email:
Computer Systems Science and Engineering 2021, 37(1), 135-148. https://doi.org/10.32604/csse.2021.014030
Received 30 August 2020; Accepted 22 September 2020; Issue published 05 February 2021
Abstract
Detecting anomaly logs is a great significance step for guarding system faults. Due to the uncertainty of abnormal log types, lack of real anomaly logs and accurately labeled log datasets. Existing technologies cannot be enough for detecting complex and various log point anomalies by using human-defined rules. We propose a log anomaly detection method based on Generative Adversarial Networks (GAN). This method uses the Encoder-Decoder framework based on Long Short-Term Memory (LSTM) network as the generator, takes the log keywords as the input of the encoder, and the decoder outputs the generated log template. The discriminator uses the Convolutional Neural Networks (CNN) to identify the difference between the generated log template and the real log template. The model parameters are optimized automatically by iteration. In the stage of anomaly detection, the probability of anomaly is calculated by the Euclidean distance. Experiments on real data show that this method can detect log point anomalies with an average precision of 95%. Besides, it outperforms other existing log-based anomaly detection methods.Keywords
Cite This Article
Citations
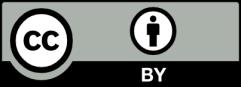