Open Access
ARTICLE
Mammogram Learning System for Breast Cancer Diagnosis Using Deep Learning SVM
1 Research Scholar, Anna University, Chennai, Tamilnadu, 600 025, India
2 Velammal Engineering College, Chennai, Tamilnadu, 600 066, India
* Corresponding Author: G. Jayandhi. Email:
Computer Systems Science and Engineering 2022, 40(2), 491-503. https://doi.org/10.32604/csse.2022.016376
Received 31 December 2020; Accepted 01 May 2021; Issue published 09 September 2021
Abstract
The most common form of cancer for women is breast cancer. Recent advances in medical imaging technologies increase the use of digital mammograms to diagnose breast cancer. Thus, an automated computerized system with high accuracy is needed. In this study, an efficient Deep Learning Architecture (DLA) with a Support Vector Machine (SVM) is designed for breast cancer diagnosis. It combines the ideas from DLA with SVM. The state-of-the-art Visual Geometric Group (VGG) architecture with 16 layers is employed in this study as it uses the small size of 3 × 3 convolution filters that reduces system complexity. The softmax layer in VGG assumes that the training samples belong to exactly only one class, which is not valid in a real situation, such as in medical image diagnosis. To overcome this situation, SVM is employed instead of the softmax layer in VGG. Data augmentation is also employed as DLA usually requires a large number of samples. VGG model with different SVM kernels is built to classify the mammograms. Results show that the VGG-SVM model has good potential for the classification of Mammographic Image Analysis Society (MIAS) database images with an accuracy of 98.67%, sensitivity of 99.32%, and specificity of 98.34%.Keywords
Cite This Article
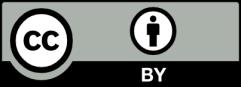