Open Access
ARTICLE
Machine Learning-Based Pruning Technique for Low Power Approximate Computing
1 Department of Electronics and Communication Engineering, Madurai Institute of Engg and Technology, Sivagangai, Tamilnadu, 630611, India
2 Pilecubes India Pvt Ltd., Chennai, Tamilnadu, India
3 Department of Electronics and Communication Engineering, Malla Reddy Engineering College (Autonomous), Secunderabad, 500100, India
4 Department of Electronics and Communication Engineering, R. M. K. Engineering College, Kavaraipettai, Chennai, Tamil Nadu, 601206, India
5 Department of Electronics and Communication Engineering, K Ramakrishnan College of Engineering, Kariyamanickam Rd, Tamil Nadu, 621112, India
* Corresponding Author: B. Sakthivel. Email:
Computer Systems Science and Engineering 2022, 42(1), 397-406. https://doi.org/10.32604/csse.2022.021637
Received 09 July 2021; Accepted 12 August 2021; Issue published 02 December 2021
Abstract
Approximate Computing is a low power achieving technique that offers an additional degree of freedom to design digital circuits. Pruning is one of the types of approximate circuit design technique which removes logic gates or wires in the circuit to reduce power consumption with minimal insertion of error. In this work, a novel machine learning (ML) -based pruning technique is introduced to design digital circuits. The machine-learning algorithm of the random forest decision tree is used to prune nodes selectively based on their input pattern. In addition, an error compensation value is added to the original output to reduce an error rate. Experimental results proved the efficiency of the proposed technique in terms of area, power and error rate. Compared to conventional pruning, proposed ML pruning achieves 32% and 26% of the area and delay reductions in 8*8 multiplier implementation. Low power image processing algorithms are essential in various applications like image compression and enhancement algorithms. For real-time evaluation, proposed ML optimized pruning is applied in discrete cosine transform (DCT). It is a basic element of image and video processing applications. Experimental results on benchmark images show that proposed pruning achieves a very good peak signal-to-noise ratio (PSNR) value with a considerable amount of energy savings compared to other methods.Keywords
Cite This Article
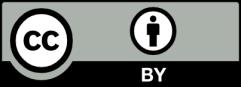