Open Access
ARTICLE
An Efficient Attention-Based Strategy for Anomaly Detection in Surveillance Video
1 Department of Computer Science and Engineering, Chung-Ang University, Seoul, 06974,
Korea
2 Intelligent Convergence Research Lab., ETRI, DaeJeon, 34129, Korea
3 Department of Electrical and Electronics Engineering, Chung-Ang University, Seoul, 06974, Korea
4 College of Art and Technology, Chung-Ang University, Anseong, 17546, Korea
* Corresponding Authors: Sangoh Park. Email: ; Sanghyun Seo. Email:
Computer Systems Science and Engineering 2023, 46(3), 3939-3958. https://doi.org/10.32604/csse.2023.034805
Received 28 July 2022; Accepted 21 November 2022; Issue published 03 April 2023
Abstract
In the present technological world, surveillance cameras generate an immense amount of video data from various sources, making its scrutiny tough for computer vision specialists. It is difficult to search for anomalous events manually in these massive video records since they happen infrequently and with a low probability in real-world monitoring systems. Therefore, intelligent surveillance is a requirement of the modern day, as it enables the automatic identification of normal and aberrant behavior using artificial intelligence and computer vision technologies. In this article, we introduce an efficient Attention-based deep-learning approach for anomaly detection in surveillance video (ADSV). At the input of the ADSV, a shots boundary detection technique is used to segment prominent frames. Next, The Light-weight Convolution Neural Network (LWCNN) model receives the segmented frames to extract spatial and temporal information from the intermediate layer. Following that, spatial and temporal features are learned using Long Short-Term Memory (LSTM) cells and Attention Network from a series of frames for each anomalous activity in a sample. To detect motion and action, the LWCNN received chronologically sorted frames. Finally, the anomaly activity in the video is identified using the proposed trained ADSV model. Extensive experiments are conducted on complex and challenging benchmark datasets. In addition, the experimental results have been compared to state-of-the-art methodologies, and a significant improvement is attained, demonstrating the efficiency of our ADSV method.Keywords
Cite This Article
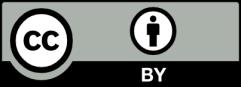