Open Access
ARTICLE
Web Intelligence with Enhanced Sunflower Optimization Algorithm for Sentiment Analysis
Department of Information Technology, College of Computer and Information Sciences, Princess Nourah bint Abdulrahman University, P.O.Box 84428, Riyadh, 11671, Saudi Arabia
* Corresponding Author: Abeer D. Algarni. Email:
Computer Systems Science and Engineering 2023, 47(1), 1233-1247. https://doi.org/10.32604/csse.2022.026915
Received 06 January 2022; Accepted 11 February 2022; Issue published 26 May 2023
Abstract
Exponential increase in the quantity of user generated content in websites and social networks have resulted in the emergence of web intelligence approaches. Several natural language processing (NLP) tools are commonly used to examine the large quantity of data generated online. Particularly, sentiment analysis (SA) is an effective way of classifying the data into different classes of user opinions or sentiments. The latest advances in machine learning (ML) and deep learning (DL) approaches offer an intelligent way of analyzing sentiments. In this view, this study introduces a web intelligence with enhanced sunflower optimization based deep learning model for sentiment analysis (WIESFO-DLSA) technique. The major intention of the WIESFO-DLSA technique is to identify the expressions or sentiments that exist in the social networking data. The WIESFO-DLSA technique initially performs pre-processing and word2vec feature extraction processes to generate a meaningful set of features. At the same time, bidirectional long short term memory (BiLSTM) model is applied for classification of sentiments into different class labels. Moreover, an enhanced sunflower optimization (ESFO) algorithm is exploited to optimally adjust the hyperparameters of the BiLSTM model. A wide range of simulation analyses is performed to report the better outcomes of the WISFO-DLSA technique and the experimental outcomes ensured its promising performance under several measures.Keywords
Cite This Article
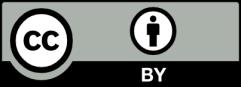