Open Access
ARTICLE
An Ensemble Machine Learning Technique for Stroke Prognosis
1 Department of Computer Science, College of Computer Engineering and Sciences, Prince Sattam bin Abdulaziz University Al-Kharj, 16273, Saudi Arabia
2 Department of Computer Science, COMSATS University, Islamabad, 53000, Pakistan
3 Computer Science Department, Faculty of Computers & Information Technology, University of Tabuk, Tabuk, 71491, Saudi Arabia
4 Information Systems Department, Faculty of Management, Comenius University in Bratislava, Odbojárov, Bratislava, 440, Slovakia
5 Department of Computer Engineering and Networks, College of Computer and Information Sciences, Jouf University, Sakaka, 72388, Saudi Arabia
6 Department of Computer Science and Software Engineering, International Islamic University, Islamabad, 44000, Pakistan
* Corresponding Authors: Mesfer Al Duhayyim. Email: ; Sidra Abbas. Email:
Computer Systems Science and Engineering 2023, 47(1), 413-429. https://doi.org/10.32604/csse.2023.037127
Received 24 October 2022; Accepted 28 December 2022; Issue published 26 May 2023
Abstract
Stroke is a life-threatening disease usually due to blockage of blood or insufficient blood flow to the brain. It has a tremendous impact on every aspect of life since it is the leading global factor of disability and morbidity. Strokes can range from minor to severe (extensive). Thus, early stroke assessment and treatment can enhance survival rates. Manual prediction is extremely time and resource intensive. Automated prediction methods such as Modern Information and Communication Technologies (ICTs), particularly those in Machine Learning (ML) area, are crucial for the early diagnosis and prognosis of stroke. Therefore, this research proposed an ensemble voting model based on three Machine Learning (ML) algorithms: Random Forest (RF), Extreme Gradient Boosting (XGBoost), and Light Gradient Boosting Machine (LGBM). We apply data preprocessing to manage the outliers and useless instances in the dataset. Furthermore, to address the problem of imbalanced data, we enhance the minority class’s representation using the Synthetic Minority Over-Sampling Technique (SMOTE), allowing it to engage in the learning process actively. Results reveal that the suggested model outperforms existing studies and other classifiers with 0.96% accuracy, 0.97% precision, 0.97% recall, and 0.96% F1-score. The experiment demonstrates that the proposed ensemble voting model outperforms state-of-the-art and other traditional approaches.Keywords
Cite This Article
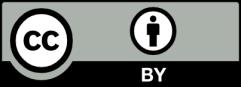