Open Access
ARTICLE
Automatic Examination of Condition of Used Books with YOLO-Based Object Detection Framework
1 Department of Industrial Engineering, Seoul National University of Science and Technology, Seoul, 01811, Korea
2 Department of Data Science, Seoul National University of Science and Technology, Seoul, 01811, Korea
* Corresponding Author: Jin-Woo Jeong. Email:
Computer Systems Science and Engineering 2023, 47(2), 1611-1632. https://doi.org/10.32604/csse.2023.038319
Received 07 December 2022; Accepted 25 April 2023; Issue published 28 July 2023
Abstract
As the demand for used books has grown in recent years, various online/offline market platforms have emerged to support the trade in used books. The price of used books can depend on various factors, such as the state of preservation (i.e., condition), the value of possession, and so on. Therefore, some online platforms provide a reference document to evaluate the condition of used books, but it is still not trivial for individual sellers to determine the price. The lack of a standard quantitative method to assess the condition of the used book would confuse both sellers and consumers, thereby decreasing the user experience of the online secondhand marketplace. Therefore, this paper discusses the automatic examination of the condition of used books based on deep learning approaches. In this work, we present a book damage detection system based on various You Only Look Once (YOLO) object detection models. Using YOLOv5, YOLOR, and YOLOX, we also introduce various training configurations that can be applied to improve performance. Specifically, a combination of different augmentation strategies including flip, rotation, crop, mosaic, and mixup was used for comparison. To train and validate our system, a book damage dataset composed of a total of 620 book images with 3,989 annotations, containing six types of damages (i.e., Wear, Spot, Notch, Barcode, Tag, and Ripped), collected from the library books is presented. We evaluated each model trained with different configurations to figure out their detection accuracy as well as training efficiency. The experimental results showed that YOLOX trained with its best training configuration yielded the best performance in terms of detection accuracy, by achieving 60.0% (mAP@.5:.95) and 72.9% (mAP@.5) for book damage detection. However, YOLOX performed worst in terms of training efficiency, indicating that there is a trade-off between accuracy and efficiency. Based on the findings from the study, we discuss the feasibility and limitations of our system and future research directions.Keywords
Cite This Article
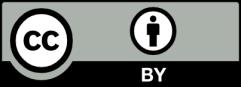