Open Access
ARTICLE
SlowFast Based Real-Time Human Motion Recognition with Action Localization
1 Department of Computer Science, Kyonggi University, Suwon, 16227, Korea
2 Contents Convergence Software Research Institute, Kyonggi University, Suwon, 16227, Korea
3 Division of AI Computer Science and Engineering, Kyonggi University, Suwon, 16227, Korea
* Corresponding Author: Kyungyong Chung. Email:
Computer Systems Science and Engineering 2023, 47(2), 2135-2152. https://doi.org/10.32604/csse.2023.041030
Received 08 April 2023; Accepted 23 May 2023; Issue published 28 July 2023
Abstract
Artificial intelligence is increasingly being applied in the field of video analysis, particularly in the area of public safety where video surveillance equipment such as closed-circuit television (CCTV) is used and automated analysis of video information is required. However, various issues such as data size limitations and low processing speeds make real-time extraction of video data challenging. Video analysis technology applies object classification, detection, and relationship analysis to continuous 2D frame data, and the various meanings within the video are thus analyzed based on the extracted basic data. Motion recognition is key in this analysis. Motion recognition is a challenging field that analyzes human body movements, requiring the interpretation of complex movements of human joints and the relationships between various objects. The deep learning-based human skeleton detection algorithm is a representative motion recognition algorithm. Recently, motion analysis models such as the SlowFast network algorithm, have also been developed with excellent performance. However, these models do not operate properly in most wide-angle video environments outdoors, displaying low response speed, as expected from motion classification extraction in environments associated with high-resolution images. The proposed method achieves high level of extraction and accuracy by improving SlowFast’s input data preprocessing and data structure methods. The input data are preprocessed through object tracking and background removal using YOLO and DeepSORT. A higher performance than that of a single model is achieved by improving the existing SlowFast’s data structure into a frame unit structure. Based on the confusion matrix, accuracies of 70.16% and 70.74% were obtained for the existing SlowFast and proposed model, respectively, indicating a 0.58% increase in accuracy. Comparing detection, based on behavioral classification, the existing SlowFast detected 2,341,164 cases, whereas the proposed model detected 3,119,323 cases, which is an increase of 33.23%.Keywords
Cite This Article
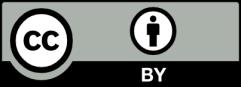