Open Access
ARTICLE
Deep Learning Based Vehicle Detection and Counting System for Intelligent Transportation
1 Department of Computer Science and Engineering (Data Science), Sri Venkateshwara College of Engineering and Technology (Autonomous), Chittoor, Andhra Pradesh 517127, India
2 Department of Computational Intelligence, SRM Institute of Science and Technology, Tamil Nadu, 603203, India
3 Department of Computer Science, College of Computer Engineering and Sciences, Prince Sattam Bin Abdulaziz University, P.O.Box. 151, Alkharj, 11942, Saudi Arabia
4 University Center for Research and Development (UCRD), Department of Computer Science and Engineering, Chandigarh University, Gharuan, Mohali, Punjab, 140413, India
5 Department of Computer Science, St.Antony’s College of Arts and Sciences for Women (Affiliated to Mother Teresa Women's University), Dindigul, 624005, India
6 Department of Applied Data Science, Noroff University College, Kristiansand, Norway
7 Artificial Intelligence Research Center (AIRC), College of Engineering and Information Technology, Ajman University, Ajman, United Arab Emirates
8 Department of Electrical and Computer Engineering, Lebanese American University, Byblos, Lebanon
9 Department of Software, Kongju National University, Cheonan, 31080, Korea
* Corresponding Author: Jungeun Kim. Email:
Computer Systems Science and Engineering 2024, 48(1), 115-130. https://doi.org/10.32604/csse.2023.037928
Received 21 November 2022; Accepted 02 February 2023; Issue published 26 January 2024
Abstract
Traffic monitoring through remote sensing images (RSI) is considered an important research area in Intelligent Transportation Systems (ITSs). Vehicle counting systems must be simple enough to be implemented in real-time. With the fast expansion of road traffic, real-time vehicle counting becomes essential in constructing ITS. Compared with conventional technologies, the remote sensing-related technique for vehicle counting exhibits greater significance and considerable advantages in its flexibility, low cost, and high efficiency. But several techniques need help in balancing complexity and accuracy technique. Therefore, this article presents a deep learning-based vehicle detection and counting system for ITS (DLVDCS-ITS) in remote sensing images. The presented DLVDCS-ITS technique intends to detect vehicles, count vehicles, and classify them into different classes. At the initial level, the DLVDCS-ITS method applies an improved RefineDet method for vehicle detection. Next, the Gaussian Mixture Model (GMM) is employed for the vehicle counting process. Finally, sooty tern optimization (STO) with a deep convolutional autoencoder (DCAE) model is used for vehicle classification. A brief experimental analysis was made to demonstrate the enhanced performance of the DLVDCS-ITS technique. The comparative analysis displays the DLVDCS-ITS method's supremacy over the current state-of-the-art approaches.Keywords
Cite This Article
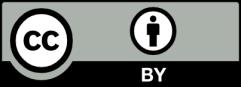