Open Access
ARTICLE
Study on Comprehensive Efficiency Evaluation of Rural Power Grid under Rural Revitalization Strategy Considering Regional Differences
1 School of Economics and Management, North China Electric Power University, Beijing, 102206, China
2 Integrated Institute for Grid Development, State Grid Energy Research Institute Co., Ltd., Beijing, 102209, China
3 Development Department, State Grid Fujian Electric Power Co., Ltd., Fuzhou, 350003, China
4 Energy Development Research Center, Economic and Technical Research Institute of State Grid Fujian Electric Power Co., Ltd., Fuzhou, 350013, China
* Corresponding Author: Manyu Yao. Email:
Energy Engineering 2023, 120(10), 2211-2231. https://doi.org/10.32604/ee.2023.029371
Received 14 February 2023; Accepted 27 April 2023; Issue published 28 September 2023
Abstract
As an essential infrastructure, the rural power grid is vital in promoting agricultural and rural carbon sequestration and improving rural energy electrification. It is necessary to carry out in-depth research on its comprehensive efficiency. Based on the requirements of “double carbon” and rural revitalization strategy for the rural Power Grid, this paper focuses on the modernization and low-carbon transformation of the rural Power Grid. It constructs an input-output index system for the investment efficiency of the rural Power Grid in China under the new situation. It uses the primary data of the rural Power Grid of 30 prefecture-level cities in China from 2019 to 2021 to evaluate the investment efficiency of the rural Power Grid in China through a three-stage DEA model. The empirical results show that: considering the regional differences, the comprehensive efficiency of the rural Power Grid in backward areas is significantly improved; that is, the three-stage DEA method can more objectively evaluate the comprehensive efficiency of the rural Power Grid. In the context of the continued promotion of the rural revitalization strategy, to improve the comprehensive efficiency of the rural Power Grid, it is necessary to improve the technical level of the rural Power Grid further and appropriately expand the scale of investment in the rural Power Grid.Keywords
Climate change is currently a scientific and political issue of great global concern. Excessive emissions of greenhouse gases have led to a growing greenhouse effect, which hurts the global climate, and carbon dioxide is an essential part of greenhouse gases; reducing its emissions is considered the most critical way to solve the climate problem, and how to reduce carbon emissions has become a global issue. China is the most significant carbon emitter today, and the power industry is the largest single industry in China in terms of total carbon emissions. There are still problems, such as unreasonable energy consumption structure and low energy consumption levels in rural municipalities in China. Agriculture is an important source of greenhouse gas emissions [1]; the Chinese government attaches importance to the issue of carbon emissions and has introduced a series of policies to promote carbon emission reduction in rural areas. In October 2021, the State Council issued the Notice on the Action Plan for Carbon Peaking by 2030, proposing to strengthen the construction of rural power grids, improve rural energy electrification, and promote agriculture. In February 2023, the State Council promulgated the Opinions on the Key Efforts to Comprehensively Promote Rural Revitalization in 2023, which promotes the consolidation and upgrading of rural power grids and the development of rural renewable energy. Upgrading rural power grids can enhance the power supply guarantee capacity in rural areas, improve the level of rural electrification and promote clean energy development in rural areas. However, the efficiency of the rural network is affected by many factors, which are difficult to measure scientifically, making it difficult to make accurate investment planning based on the efficiency of the rural network. Therefore, in the context of “double carbon” and rural revitalization, the investment efficiency of rural power grids, as an essential infrastructure, is receiving increasing attention.
There have been abundant studies on the efficiency benefits of power grid projects. However, they mainly focus on transmission and urban distribution grids [2–5], and there needs to be more literature evaluating rural power grid efficiency. In terms of evaluation indexes, relevant studies analyze the comprehensive efficiency benefits of power grids in terms of economic benefits, social benefits, and operational efficiency. For example, the literature [6] combined the characteristics of power grid enterprises to build a comprehensive benefit evaluation system containing economic, environmental, social, and technical safety benefits. Literature [7] constructed a new rural Power Grid investment evaluation system from 4 dimensions: project effectiveness, management effectiveness, energy saving, emission reduction, and value-added services. The literature [8] established a comprehensive evaluation index system of distribution network effectiveness containing 35 indicators in six dimensions: reliability, power quality, economy, environmental protection, interactivity, and technology. The literature [9] proposed an evaluation index system of grid investment efficiency effectiveness containing incremental inputs, stock inputs, and output efficiency benefits. The literature [10] constructed a new distribution network investment efficiency evaluation index system based on the characteristics of distribution network investment under a high proportion of renewable energy from three dimensions: power supply guarantee capacity, total carrying capacity, and value creation capacity. The literature [11] considered distributed power access and customer-side load and establishes a comprehensive evaluation system for rural power grids containing 19 indicators in three dimensions: power, grid, and load. The literature [12] combined the characteristics of current rural grid construction and renovation projects to establish a rural grid characteristic index system containing 14 secondary indicators of rural grid economy, attributes, and security. The literature [13] proposed a new rural grid evaluation system including power supply capacity, operation effect, and investment effect.
In terms of evaluation methods, related scholars mainly use multi-attribute decision-making [14,15], financial analysis [16], techno-economic analysis [17], and optimization theory [18,19] to study the efficiency and effectiveness of power grid projects. Among them, multi-attribute decision-making methods can reflect the efficiency level of power grid engineering more comprehensively and thus have received attention from scholars, such as fuzzy comprehensive evaluation [20], object meta-topology [21], TOPSIS [22], ELECTRE [23], MARCOS [24], MABAC [25], AHP [26] and VIKOR [27]. Multi-attribute decision-making also has disadvantages: the index system mainly focuses on the project’s output, such as economic and social output. Although some literature considers both input and output indicators, it is not easy to make precise judgments on the efficiency of the project from the perspective of input and output correlation and applying value engineering principles. In addition, data envelopment analysis, first proposed by three operations researchers, Charnes, Cooper, and Rhodes, in 1978, has been widely used in recent years as a powerful tool for energy efficiency analysis and has now become a well-established method for measuring the efficiency of grid engineering [28]. For example, literature [29] proposed a novel network DEA perspective cross-efficiency model by considering the performance of generation and transmission systems, and literature [30] adopted an efficiency evaluation method combining the multi-DEA model and Gini criterion to comprehensively assess the reasonableness of operation and maintenance costs of local grid companies in 26 provinces, municipalities and autonomous regions in China. The literature [31] established an incremental distribution grid efficiency assessment model based on the entropy method and DEA. Previous scholars have also conducted numerous studies on rural grid efficiency using DEA methods [32,33]; for example, literature [34] used the DEA model, super-efficient DEA model, and dynamic DEA model to comprehensively assess the investment efficiency of G rural grid from 2012–2014. The literature [35] used two-stage data envelopment analysis (DEA) to calculate the efficiency assessment of county-level rural grid data from 2006–2020.
However, although using the DEA method considers the inputs and outputs from the value engineering perspective, it only sufficiently considers environmental factors other than engineering inputs and outputs (e.g., external economic and social development level), which may lead to biased efficiency assessment results. The above research provides some reference for evaluating rural power grid investment efficiency from indicators and methods in this paper.
Overall, the main contribution of this paper is twofold. First, we consider the requirements of modernization and decarbonization of rural agriculture for rural power grids in the context of “double carbon” and rural revitalization and construct a set of indicators that can reflect the new connotation of rural power grid investment efficiency. Secondly, considering the development differences between different regions, we propose a three-stage DEA model-based assessment method for rural grid investment efficiency and conduct an empirical analysis with the rural grid data of 30 prefecture-level cities in China in the past three years.
The rest of this paper is organized as follows: Section 2 constructs an index system for evaluating the grid investment efficiency of rural grids; Section 3 introduces a three-stage DEA-based model for evaluating the investment efficiency of rural grids; Section 4 shows the results of the empirical analysis and the applicability analysis of the model; Section 5 concludes the paper with corresponding policy recommendations and subsequent outlook.
2 Rural Grid Input-Output Indicator Set
In order to achieve the “double carbon” target, by 2025, the proportion of non-fossil energy consumption in China needs to reach about 20%. At the same time, in the context of the rural revitalization strategy, promoting emission reduction and carbon sequestration in agriculture and rural areas requires vigorously promoting the development and utilization of renewable energy in rural areas and supporting the development of new modes and new business models of rural energy. The realization of the above goals depends on a solid and intelligent rural power grid infrastructure, so it is urgent and necessary to accelerate the rural power grid consolidation and upgrading project and improve the efficiency of rural power grid investment.
Based on this, this paper takes the requirements of “double carbon” and rural revitalization strategy for the rural Power Grid as the target orientation, focuses on the direction of modernization and low carbon transformation of the rural Power Grid, and constructs an input-output index system to reflect the investment efficiency of the rural Power Grid in China under the new situation, as shown in Table 1, which contains three input indicators, four output indicators, and three environmental indicators.
(1) Input indicators. Scholars have conducted many studies on the input indicators of rural power grids and believe that the investment amount of the rural Power Grid reflects the scale condition of the local rural grid. The leading equipment of rural distribution network operations is transformers and lines [34]. Therefore, this paper, drawing on the previous research results [30,35], uses annual investment operation and maintenance fee, line length, and transformer capacity as input variables to reflect the scale of the rural grid.
(2) Output indicators. Rural power grids are of great significance to rural economic and social development, such as the rural wells electricity project and household electricity project, which have significant social benefits, so the output indicators need to take into account the social benefits based on the economic benefits of rural Power Grid projects [7]. The rural revitalization strategy puts forward many requirements for the rural Power Grid, such as ensuring the production and living of rural residents with electricity, improving the level of rural infrastructure, promoting the development of rural industrialization, and promoting the transformation of rural energy. The selection of output indicators has been extensively studied by relevant scholars [11,36]. Therefore, this paper selects the Reliability rate of rural Power Grid power supply, rural electrification rate, the annual increase in power supply per unit investment, and clean energy penetration rate as output variables.
(3) External environmental indicators. The per capita disposable income of rural residents refers to the part of the total cash income of rural households that can be used to arrange the household’s daily life. The per capita consumption expenditure of rural residents refers to the total expenditure of rural residents to meet the daily consumption of the household, both of which can reflect the economic development momentum of rural areas [37]; the gross value of agriculture, forestry, animal husbandry, and fishery is one of the leading indicators of the agricultural statistical system, which from the value perspective It reflects the full scale and level of agricultural production. Its composition reflects the distribution characteristics of the agricultural industry structure. Therefore, this paper selects three factors, including per capita disposable income of rural residents, gross agricultural, forestry, animal husbandry, and fishery products, and per capita consumption expenditure of rural residents, as environmental variables.
3 A Three-Stage DEA-Based Rural Grid Efficiency Assessment Model
Data Envelopment Analysis (DEA) is a multidimensional assessment method that incorporates multiple input and output indicators and can utilize linear programming techniques to handle multiple input and output variables simultaneously without needing a cost function. This feature makes it a suitable tool for assessing the relative efficiency of projects [38,39]. Compared with other algorithms, DEA has the following applicability in rural grid efficiency assessment: first, it does not need to make weight assumptions when studying the production function theory of multiple inputs and outputs, and it does not need to determine the explicit function relationship between inputs and outputs in advance, which significantly improves the objectivity of rural grid efficiency assessment results; second, the optimal benefit value of DMU is independent of the selection of the magnitudes of input and output index values, which can Third, DEA cannot only realize quantitative assessment but also give the specific difference amount of each index, which can help guide the rural grid in each region to realize the improvement of grid investment efficiency value by adjusting the number of inputs or outputs. Moreover, three-stage DEA can eliminate the influence of external environmental factors and random disturbances on rural grid efficiency measurement on this basis and reflect the investment efficiency situation of the rural grid in each city more reasonably.
The specific process is as follows:
(1) Phase I: Traditional DEA model
In this paper, according to the research problem, an input-oriented variable payoff of scale model (DEA-BCC) is used to evaluate the integrated efficiency of rural power grids. The specific BCC model is as follows:
where,
(2) Second stage: stochastic frontier model SFA
With the help of the SFA model to eliminate the influence of random errors and environmental factors on efficiency estimation, the adjusted optimal input values are finally derived, and the specific SFA regression model is shown as follows:
where,
The results obtained from the regression model, in order to adjust the inputs of each decision unit, corresponding to the following formula:
where,
By excluding the effects of random errors and environmental factors on the efficiency estimation of the decision unit, the rural grids in the sample areas are all placed in the same external environment to obtain the input redundancy caused by management inefficiency only.
(3) Stage 3: Adjusted DEA model with inputs
The DEA-BCC model is used to calculate the comprehensive efficiency of the rural power grid in the sample area again, with the new input variable adjusted by the SFA model in the second stage and the original output variable, to obtain an accurate and objective value of rural power grid investment efficiency.
In summary, the process of using a three-stage DEA to carry out a comprehensive rural grid efficiency assessment is shown in Fig. 1.
Figure 1: Integrated rural grid efficiency assessment process
4 Empirical Analysis and Discussion
4.1 Analysis of Empirical Objects
Considering the availability of raw data, this paper compiles raw input, output, and external environment variable data for rural power grids in 30 prefecture-level cities in China from 2019 to 2021. The external environment data of each decision unit are shown in the Appendix A. Referring to the World Bank’s definition of developed and developing countries, this paper divides the decision units into two categories, developed and developing regions, by whether the GDP per capita of the region reaches the average level, as shown in Table 2. The descriptive statistics of the input-output variable data are shown in Table 3.
Use DEAP2.1 software to calculate the investment efficiency of the rural power grid and DEA calculations for the first stage of 30 DMUs, as shown in Table 4.
The first stage of DEA results preliminarily shows the investment efficiency of rural power grids in 30 prefecture-level cities from 2019 to 2021. Without stripping off the influence of environmental factors and random disturbances, the average investment efficiency of rural power grids in these three years is 0.856, 0.889, and 0.898, respectively, indicating that under the existing investment conditions, the investment efficiency of rural power grids still has some room for improvement.
Through the effectiveness of DEA, 7, 14, and 14 municipalities remained on the efficiency frontier surface in 2019–2021. Three municipalities are on the efficiency frontier surface in all three years, namely DMU13, DMU15, and DMU25, and the investment efficiency of rural grids in these three municipalities has been on the frontier surface with the best efficiency in all aspects; the pure technical efficiency of rural grids in some municipalities is 1. For example, the pure technical efficiency of DMU11 is 1 for three consecutive years, but its scale efficiency is lower than the overall average level, resulting in its inability to be at the frontier of production; in some developing regions represented by DMU17, its rural grid investment efficiency, and pure technical efficiency are lower than the sample regions, indicating that the efficiency of all aspects of the rural grid in these regions still needs to be improved.
In general, the investment efficiency of Rural Grid is improving. However, this measurement does not exclude the influence of environmental and stochastic perturbations. It needs to accurately show the investment efficiency of the Rural Grid in each municipality, so further adjustment and measurement are needed.
The input slack variables (explanatory variables) isolated in the previous stage are regressed using a stochastic frontier (SFA) model, in which the slack variables include three input variables selected in this paper, such as annual investment operation and maintenance fees, transformer capacity and line length and three external environmental variables, such as per capita disposable income of rural residents, the gross output value of agriculture, forestry, animal husbandry, and fishery, and per capita consumption expenditure of rural residents in the selected localities, are used as explanatory variables. The specific SFA regression results are shown in Table 5.
As shown in Table 4, the one-sided tests of the SFA model corresponding to the three input slack variables all pass the 1% significance test, indicating that the SFA regression is valid and the regression model is correctly defined. The value for all input variables is 1, which further indicates that management inefficiency is the main factor causing the investment inefficiency of the decision unit. The coefficients of each input variable are significant at the 1% level, indicating that the environmental variables significantly affect the overall efficiency of the rural grid in the sample municipalities.
Due to the differences in the coefficients of the slack variables for each year, the analysis is conducted here with 2019 as an example. From the analysis of the regression results, it can be seen that: the impact of rural residents’ per capita disposable income on the slack variables of annual investment operation and maintenance fee and line length is significantly both negative, indicating that the per capita disposable income of rural residents is conducive to the optimization of resource allocation in the rural grid and the improvement of the comprehensive efficiency of the rural Power Grid; the impact of rural residents’ per capita consumption expenditure on the slack variables of annual investment operation and maintenance fee and line length is significantly positive, indicating that the per capita consumption expenditure of rural residents increases will lead to redundancy of rural grid inputs with a low degree of impact.
Eliminating the effects of environmental factors and random disturbances on the efficiency measurement of rural grids, adjusting the original input variables, and then using the DEA model for efficiency assessment can more reasonably reflect the investment efficiency of rural grids, and the results are shown in Table 6.
The analysis results comparing the first and third stages are shown in Table 7. It is found that after excluding the influence of external environmental factors and random disturbance terms, the investment efficiency of rural power grids in each city improved between 2019 and 2021, and the mean value of investment efficiency increased from 0.856, 0.889, and 0.898 to 0.904, 0.908, and 0.920 respectively in these three years; the standard deviation of investment efficiency in each year increases from 0.134, 0.143, and 0.121 to 0.102, 0.13, and 0.12, respectively, i.e., after the third stage of adjustment, the comprehensive efficiency of rural Power Grid investment improves, and the difference decreases. 12, 17, and 18 municipalities remain at the forefront of efficiency in 2019–2021, respectively, and the investment efficiency values in most regions improve significantly compared with the first stage. The adjusted mean values of pure technical efficiency of the rural grid are at [0.945, 0.971], and the mean values of scale efficiency are at [0.935, 0.971], and both of their efficiency means have increased. The results indicate that the traditional DEA model needs to accurately show the investment efficiency of the rural grid in each municipality. Its calculation underestimates the investment efficiency of rural grids without considering environmental factors and random disturbances.
Since the changes in efficiency values are more evident in 2019, the year 2019 is used as an example here for specific analysis. Analysis of Table 6 shows that: in the first stage of assessment, the efficiency values of rural grids in developing regions such as DMU8 and DMU12 are underestimated, and they all reach the frontier level after excluding the development differences between municipalities; on the contrary, DMU15 in developed cities does not reach the frontier level in the third stage, and the technical efficiency of DMU2 and DMU20 in developed cities is overestimated in the first stage, indicating that the three-stage DEA can take into account to the development differences between local cities, thus deriving a more realistic investment efficiency. In the third stage, the pure technical efficiency value of six municipalities, such as DMU1 and DMU4 is 1. The reason for not reaching the comprehensive efficiency frontier is the low scale efficiency, among which the scale efficiency value of DMU9 is 0.793, and the scale efficiency can be improved by increasing the investment amount and strengthening infrastructure construction; in addition, the scale efficiency values of two municipalities such as DMU3 and DMU21 are 1, and these municipalities The main reason why these municipalities are not at the forefront of investment efficiency is their low pure technical efficiency, which indicates that the technology and management level of the local rural grid is low. Investing in the rural grid can be made efficient by upgrading technology and improving management.
The mean values of the third-stage efficiency results from 2019–2021 are shown in Fig. 2. From the time dimension, the average comprehensive efficiency value increases from 0.904 to 0.920, showing an upward trend. The analysis of the reasons shows that the government attaches importance to developing rural power grids, continuously increases the financial investment in rural power grids, and promotes the construction of rural power grid infrastructure, and the construction of rural power grids has achieved positive results. The average pure technical efficiency rises and then falls, and is at the maximum value of 0.971 in 2020, and then falls to 0.945; while the average scale efficiency falls and then rises, and is 0.935 in 2020, and then rises to the maximum value of 0.971. The reason for this is that in 2020, the investment in the rural grid focused on upgrading the internal technology and improving the management level, neglecting the development of the rural grid construction scale; in 2021, on the contrary, it paid attention to the improvement of rural grid construction scale, but neglects the simultaneous improvement of technical management level. The infrastructure construction and technical management level of the rural grid needs to be upgraded simultaneously to improve the overall efficiency of the rural grid effectively.
Figure 2: Histogram of average efficiency results for phase 3, 2019–2021
In summary, the average value of comprehensive efficiency in 2021 is 0.920. Only 8% of the inputs have yet to produce benefits. However, in the three years 2019–2021, there are Seven municipalities with a comprehensive efficiency of 1, accounting for about 13% of the presidential measurement; that is, the overall comprehensive efficiency of the selected decision-making unit has not reached DEA effective, and if we want to achieve high efficiency and high quality in Rural power grid investment, we should improve the technology and management level the same time appropriately expand the scale of investment.
4.5 Model Adaptability Analysis
In order to verify the applicability of the three-stage DEA method in rural power grid efficiency evaluation, this paper makes Spearman and Pearson correlation calculation and ttest on the efficiency terms obtained from the first and third-stage DEA models, and the results are shown in Table 8.
Analysis of Table 8 reveals that: according to Spearman and Pearson correlation coefficient analysis, it can be seen that the three-stage DEA model is derived from the traditional DEA model, and the two are highly correlated; according to the t-test results, the differences between rural grid investment efficiency, pure technical efficiency, and scale efficiency are significant, indicating that after considering the development differences between municipalities, the data of the third stage and the first stage are still independent, reflecting This shows that the data of the third stage are still independent of the first stage after considering the development differences among municipalities, which reflects the effectiveness of the three-stage DEA model.
In summary, compared with the traditional DEA model, the three-stage DEA model can fully consider the influence of external environmental factors and has better applicability and validity for assessing the investment efficiency of rural power grids. The assessment results are more objective and accurate.
5 Conclusions and Policy Suggestions
The rural power grid renovation and upgrading projects are essential for people’s livelihood, which is of great significance for China to continuously promote the rural revitalization strategy and build a new rural power grid to meet the “double carbon goal”. In this context, as an essential infrastructure, conducting an in-depth study on the investment efficiency of rural power grids is especially necessary. Based on the requirements of “double carbon” and rural revitalization strategy for rural power grids, this paper constructs an input-output index system for the investment efficiency of rural power grids in China under the new situation. It measures the investment efficiency of rural power grids in 30 prefecture-level cities in China from 2019 to 2021 through a three-stage DEA model.
The research results show that (1) after the third stage of adjustment, the comprehensive investment efficiency of the rural power grid is improved. The difference is reduced, the number of municipalities in the efficiency frontier surface has increased, and the investment efficiency value in most areas is significantly improved compared with the first stage, that is, based on the input-output index system constructed in this paper to reflect the investment efficiency of China’s rural power grid under the new situation, the three-stage DEA method can be used to evaluate rural power grid investment efficiency more objectively. In the context of continuous promotion of rural revitalization strategy, to improve rural power grid investment efficiency, the technical level of the rural power grid should be further improved, and the investment scale of the rural power grid should be appropriately expanded. (2) Under the government’s policy measures to promote rural revitalization, the overall investment efficiency of China’s rural power grid has been improved, and the construction of the rural power grid has achieved positive results. The correlation coefficient analysis and t-test results show that the three-stage DEA model and the traditional DEA model are highly correlated and independent of each other. The three-stage DEA model has better applicability and validity for assessing the investment efficiency of rural power grids, and the assessment results are more objective and accurate. (3) Increasing the per capita disposable income of rural residents is conducive to optimizing the allocation of rural power grid resources and promoting the comprehensive efficiency of the rural power grid; the improvement of the development level of agriculture, forestry, animal husbandry, and fishery helps optimize the efficiency of rural power grid operation and equipment use.
Based on the above conclusions, combined with the characteristics of China’s rural power grid and the problems generally faced by power grid enterprises, we propose the following policy recommendations to improve the efficiency of investment in agricultural power grids:
(1) Increase the investment in the rural power grid. In recent years, the construction and renovation of rural power grids in China have achieved significant results, with continuous improvement in power supply reliability and significant enhancement of grid structure. However, rural power grids still need more power supply capacity, poor power supply quality, reliability, and other problems compared to urban distribution networks. Therefore, the government must increase the financial investment and policy support for rural power grids, solve some of the weaknesses of rural power grids, appropriately expand the scale of rural power grids, and improve the level of technology and intelligence of rural power grids, to effectively improve the reliability, adaptability, and economy of rural power grids and provide strong support for the “rural revitalization strategy”.
(2) Establish a long-term mechanism for rural grid development. Because of the high investment demand and low income of rural power grid, the high cost of power grid renovation seriously restricts the development of power grid enterprises, so it is necessary to continue to play the role of the main channel of national investment and establish and improve the sustainable development mechanism of the rural power grid by the state. On the one hand, financial investment, loans, taxation, and other aspects increase the policy support for rural grid development; on the other hand, a timely introduction of a new electricity price policy should ease the contradiction of rural grid renovation and upgrading project investment repayment.
(3) The implementation of rural power grid differentiated development. Considering the regional differences and development characteristics of rural areas, rural power grids’ construction and transformation requirements are different. For the rural grid infrastructure in relatively backward areas to build a safe and reliable modern rural grid, the investment direction is to improve the network structure, enhance the power supply guarantee capacity, and improve the level of power supply services; for the rural grid infrastructure is better areas to build a green low-carbon modern rural grid, the investment direction is to improve the level of grid intelligence, improve the power supply service capacity, improve the level of electrification, promote clean The investment direction is to improve the intelligence level of the power grid, improve the power supply service capacity, enhance the electrification level and promote clean energy consumption.
Although the model in this paper is applicable for assessing the investment efficiency of rural power grids, it still has some limitations. In the future, we can further consider regional differences and select environmental variables more scientifically and reasonably to improve the reliability of the conclusions. At the same time, the traditional DEA can be extended by considering the uncertainty factors in the assessment of investment efficiency of rural Power Grid to improve the generalizability and robustness of the model.
Acknowledgement: Thanks are due to the editors and reviewers for their valuable opinions, which are of great help to improve the quality of this paper.
Funding Statement: This Study is supported by the State Grid Science and Technology Project (1300-202157362A-0-0-00).
Author Contributions: Study conception and design: Huiru Zhao, Manyu Yao; data collection: Zhenqi Bai; results analysis and interpretation: Manyu Yao, Yue Zhang; manuscript preparation: Manyu Yao, Zhihua Ding, Zhenda Hu.
Availability of Data and Information: The supporting data for the findings were obtained from the State Grid Science and Technology Programme and are not available to the public. The data provided in the appendix are from the China Statistical Yearbook.
Conflicts of Interest: The authors declare that they have no conflicts of interest to report regarding the present study.
References
1. Wang, X. T., Zhang, J. B. (2022). The primary path and system construction of agricultural green and low-carbon development under the dual-carbon strategic goal. Chinese Journal of Eco-Agriculture, 30(4), 516–526. [Google Scholar]
2. Wang, Z. Q., Liu, Y. B., Shen, X. D., Yu, T., Li, J. et al. (2021). Risk constrained investment portfolio model for distribution network projects with efficiency optimization. Power System Technology, 45(1), 216–226. [Google Scholar]
3. Pan, X., Shao, J. W., Liu, S. Q., Pan, X. Y. (2022). Accurate investment strategy for urban distribution network based on grid planning. Smart Power, 50(2), 1–8. [Google Scholar]
4. Wang, R. H., Du, Y., Gou, Q. F., Yang, J., You, W. Y. et al. (2020). Evaluation of planning investment efficiency and benefit of provincial power grid considering the reform transmission and distribution prices. Electric Power Construction, 41(11), 135–144. [Google Scholar]
5. Li, Y. J., Wang, X. T., Kang, H. R., Zhang, X. L., Zhang, W. et al. (2021). A preferential model for investment in distribution networks with renewable energy considering efficiency, effectiveness and carbon emission reduction improvement. Renewable Energy Resources, 39(12), 1662–1668. [Google Scholar]
6. Du, Y., Ma, T., Wang, Q., Hu, L., Wu, G. (2020). The evaluation of power grid projects’ investment based on the reform of transmission and distribution price. Earth and Environmental Science, 440(3), 032131. [Google Scholar]
7. Wang, L. L., Jia, M. Y., Han, S., Li, M., Liu, W. (2020). Comprehensive evaluation of investment efficiency and risk capacity of rural power grids based on TOPSIS-Grey correlation degree. Journal of Electric Power Science and Technology, 35(4), 76–83. [Google Scholar]
8. Liang, H. F., Liu, Z. Y. (2023). Comprehensive benefit evaluation of smart distribution network based on analytic hierarchy process-entropy method-fuzzy comprehensive analysis. Journal of North China Electric Power University, 50(1), 48–55. [Google Scholar]
9. Peng, D. X., Dong, S. B., Wang, L. (2019). Research on efficiency and benefits evaluation system of power grid investment based on high-quality development. Construction Economy, 40(12), 107–114. [Google Scholar]
10. Li, L. J., Xie, G. L., Lin, K. Y., Hong, J. H., Ma, W. Z. et al. (2022). Investment efficiency assessment of distribution network for the high proportion of renewable energy: A hybrid multiattribute decision-making method. Mathematical Problems in Engineering, 2022. [Google Scholar]
11. Jia, M. Y., Li, M., Han, S., Li, Q. Y. (2020). Research on a comprehensive evaluation system for rural power grids based on game theory portfolio empowerment. Journal of Electric Power Science and Technology, 35(2), 69–75. [Google Scholar]
12. Zhang, Z. Z., Li, H. (2021). Construction of agricultural network characteristic index system based on principal component analysis. Industrial & Science Tribune, 20(2), 41–43. [Google Scholar]
13. Zhang, Q., Jia, M. Y. (2019). A study on investment allocation decision of rural power grid based on neural network evaluation. Power Systems and Big Data, 22(9), 72–78. [Google Scholar]
14. Li, L., Yang, D. S., Yu, M. T., Li, Z., Xiong, W. et al. (2021). Research on reliability assessment method of power grid operation based on the improved hybrid method. Electrical Measurement & Instrumentation, 58(10), 74–79. [Google Scholar]
15. Elkadeem, M. R., Kotb, K. M., Ullah, Z., Atiya, E. G., Dán, A. et al. (2021). A two-stage multi-attribute analysis method for city-integrated hybrid mini-grid design. Sustainable Cities and Society, 65, 102603. https://doi.org/10.1016/j.scs.2020.102603 [Google Scholar] [CrossRef]
16. Ji, Y. H., Zhao, Y., Leng, X. B., Niu, F., Lu, Y. D. et al. (2020). Study on the financial evaluation model of international power grid investment: Based on the case of greenland project investment in the greater mekong subregion. Communication of Finance and Accounting, 2020(24), 89–93. [Google Scholar]
17. Song, Q. Y., Zheng, J. Y., Ni, S. Y., Li, T. T., Chen, H. et al. (2020). Calculation method of incremental distribution network project investment benefit based on integrated optimal energy allocation. Science Technology and Engineering, 20(36), 14961–14966. [Google Scholar]
18. Li, Y., Chang, Y. Y., Liang, X. H. (2021). Research on an optimal reconfiguration method of distribution network benefits with new energy access. Control Engineering of China, 28(5), 931–937. [Google Scholar]
19. Tan, C. X., Geng, S. P., Tan, Z. F., Wang, J., Zhou, J. H. (2021). Optimization model of investment model for grid company participation in incremental distribution network. Power System Technology, 45(11), 4375–4387. [Google Scholar]
20. Liu, W. X., Yu, L. L., Zhang, L. H., Yuan, P., Wang, Y. et al. (2020). Evaluation of power grid development level based on AHP and multi-level fuzzy comprehensive evaluation. Smart Power, 48(5), 80–85. [Google Scholar]
21. Tan, Y. D., Wen, M., Li, X. H., Zhang, D., Wang, Z. C. et al. (2021). Comprehensive benefit evaluation of transmission network projects based on improved matter element extension model. Journal of Electric Power Science and Technology, 36(6), 113–119. [Google Scholar]
22. Ai, X., Qin, J. H., Hu, H. Y., Wang, Z. D., Peng, D. et al. (2021). Comprehensive evaluation of power grid security and benefit based on BWM entropy weight TOPSIS method. Modern Electric Power, 38(1), 60–68. [Google Scholar]
23. Cheng, Z. Y., Zhu, X. H., Li, J. Q. (2022). A multi-criteria fusion decision method for grid planning and investment based on improved ELECTRE method. Electric Power, 55(11), 59–65. [Google Scholar]
24. Zhang, Z., Lin, S., Ye, Y., Xu, Z., Zhao, Y. et al. (2022). A hybrid MCDM model for evaluating the market-oriented business regulatory risk of power grid enterprises based on the Bayesian best-worst method and MARCOS approach. Energies, 15(9), 2978. https://doi.org/10.3390/en15092978 [Google Scholar] [CrossRef]
25. Zhang, H., Jiang, J., Song, M., Yong, X. (2022). Comprehensive benefit evaluation of transmission and substation project for renewable energy connection: A case in China. Energy Reports, 8, 12653–12667. https://doi.org/10.1016/j.egyr.2022.09.085 [Google Scholar] [CrossRef]
26. Zhu, T., Xie, Y. G., Zhong, S. J., Chen, W., Zhang, P. (2022). Application of fuzzy hierarchical analysis in the process of grid operation scheme selection. Electric Power, 55(8), 121–128. [Google Scholar]
27. Jiang, H., Wu, J. H., Sa, L. Y., Zhang, Q., Yao, L. et al. (2023). Comprehensive evaluation of grid structure vulnerability based on VIKOR method and complex network theory. Water Resources and Power, 41(3), 216–220. [Google Scholar]
28. Yu, D., He, X. (2020). A bibliometric study for DEA applied to energy efficiency: Trends and future challenges. Applied Energy, 268(1), 115048. https://doi.org/10.1016/j.apenergy.2020.115048 [Google Scholar] [CrossRef]
29. Zhang, R., Wei, Q., Li, A., Ren, L. (2022). Measuring efficiency and technology inequality of China’s electricity generation and transmission system: A new approach of network data envelopment analysis prospect cross-efficiency models. Energy, 246, 123274. https://doi.org/10.1016/j.energy.2022.123274 [Google Scholar] [CrossRef]
30. Xie, L., Zhou, Z. C., You, P. P., Sun, Y. K. (2020). Evaluation of cost reasonableness and spatial divergence characteristics of Chinese grid enterprises. Geographical Research, 39(2), 399–413. [Google Scholar]
31. Ren, X. J., Ye, B., Gao, C. W., Wang, B., Yang, N. et al. (2020). Incremental distribution price setting method based on entropy value method and DEA. Electric Power Construction, 41(1), 80–87. [Google Scholar]
32. Zhu, H. (2012). Research on the comprehensive assessment method of rural power grid investment benefits (Master Thesis). Zhengzhou University, China. [Google Scholar]
33. Yang, L. Q., Zhang, L., Zhang, S. Q., Lu, S. H. (2010). Application of data envelopment analysis in investment benefit evaluation of rural power network. Power System Protection and Control, 38(4), 49–53. [Google Scholar]
34. Xiao, Y. Z. (2016). Research on G agricultural network investment efficiency evaluation system based on DEA. (Master Thesis). South China University of Technology, China. [Google Scholar]
35. Tang, B., Cheng, Z. W., Li, B., Zhu, M. M., Wang, E. et al. (2020). Research on power system evaluation based on improved robust DEA model. Chinese Journal of Electron Devices, 43(2), 273–279. [Google Scholar]
36. Xiao, B., Li, P. P., Jiang, Z., Shi, Y. G., Jiao, M. X. et al. (2020). A lean approach to rural grid improvement based on ladder combination scoring. Automation of Electric Power Systems, 44(3), 220–228. [Google Scholar]
37. Yang, H. L., Sheng, W. X., Wang, J. Y., Li, L., Wang, J. L. (2015). Analysis of investment effect of agricultural network renovation and upgrading project based on fuzzy evaluation method. Advanced Technology of Electrical Engineering and Energy, 34(2), 55–60. [Google Scholar]
38. Charnes, A., Cooper, W. W., Rhodes, E. (1978). Measuring the efficiency of decision-making units. European Journal of Operational Research, 2(6), 429–444. https://doi.org/10.1016/0377-2217(78)90138-8 [Google Scholar] [CrossRef]
39. Yadav, D. V., Parmar, D., Ganguly, R., Shukla, S. (2022). Efficiency evaluation of sewage treatment plants in Delhi, India, using tolerance-based data envelope analysis. Environmental Monitoring and Assessment, 194(12), 867. https://doi.org/10.1007/s10661-022-10528-4 [Google Scholar] [PubMed] [CrossRef]
40. Jondrow, J., Knox, L. C. A., Materov, I. S., Schmidt, P. (1982). On the estimation of technical inefficiency in the stochastic frontier production function model. Journal of Econometrics, 19(2–3), 233–238. [Google Scholar]
Cite This Article
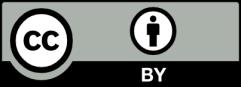