Open Access
ARTICLE
Research on Coordinated Development and Optimization of Distribution Networks at All Levels in Distributed Power Energy Engineering
1 Economic and Technological Research Institute, State Grid Hunan Electric Power Co., Ltd., Changsha, 410007, China
2 Distribution Network Planning and Research Center, Tiandi Dianyan (Beijing) Technology Co., Ltd., Beijing, 102206, China
* Corresponding Author: Guang Ouyang. Email:
Energy Engineering 2023, 120(7), 1655-1666. https://doi.org/10.32604/ee.2023.026981
Received 08 October 2022; Accepted 03 January 2023; Issue published 04 May 2023
Abstract
The uncertainty of distributed generation energy has dramatically challenged the coordinated development of distribution networks at all levels. This paper focuses on the multi-time-scale regulation model of distributed generation energy under normal conditions. The simulation results of the example verify the self-optimization characteristics and the effectiveness of real-time dispatching of the distribution network control technology at all levels under multiple time scales.Keywords
Under the continuous deepening of energy technology energy policy and electricity marketization, the distribution network must adapt to the requirements of renewable resources. The dynamic distribution network has become a way of distribution network distributed energy planning. It enables active management of large-scale distribution networks. The relatively complete grid-connected control technologies for a single distributed power source mainly include PQ control, PV control and droop control. These can not meet the dynamic control requirements of the power system. Currently, many main problems of internal combustion engines, including fluid flow, combustion, internal combustion engine performance calculation, component heat transfer and temperature field calculation, lubrication, cooling system calculation, etc., have mature software available. And these local problem models can be directly used. Scholars at home and abroad have researched distribution network planning, including distributed energy sources or charging stations. Some scholars have planned and analyzed the investment cost, active power loss and reliability of the distribution network with distributed energy sources. Reference [1] used fuzzy variables to represent load and distributed generation uncertainty. Scholars aim to minimize the unclear expected value and establish a fuzzy optimal programming model. Reference [2] applied the improved particle swarm algorithm to the problem of location and volume determination of distributed energy sources. Scholars verified the effectiveness of the model and algorithm through simulation analysis. Reference [3] studied the optimal installation location and capacity of distributed energy sources considering stochastic uncertainty.
Some scholars have established a charging station planning model considering charging characteristics, charging behavior, urban planning, transportation and power network, etc. Reference [4] proposed the optimal location and constant capacity model of wind power and photovoltaics for grid-connected electric vehicles and then uses Monte Carlo simulation to simulate. Reference [5] established a distribution network planning model considering the optimization of charging station layout but did not consider the uncertainty of renewable energy. Reference [6] thought constraints such as setting and discharging efficiency and power balance of electric vehicle swapping stations and proposed a unit combination optimization model but did not consider factors such as the environment. Reference [7] takes the minimization of system cost and pollution discharge as the optimization goal. The author fully considers demand response, energy storage, wind power, and photovoltaic-related links to establish a "source-grid-load-storage" coordination scheduling model. It adopts a multiobjective particle swarm optimization algorithm to solve it. Reference [8] comprehensively considered distributed energy, energy storage, and flexible loads to establish a multiobjective optimal scheduling model related to renewable energy utilization, network loss, and user satisfaction. The improved particle swarm algorithm is used for centralized optimization. Reference [9] analyzed the feasibility and economy of connecting electric vehicles to the grid and optimized the optimal charging and discharging of electric vehicles. This allows more EVs to be plugged into the grid, which can be charged at a lower cost. Reference [10] integrated the load into the dispatching scope into a hybrid control system for the wind and solar output accommodation problem. Scholars take optimal economic dispatch as the objective function and combine intelligent optimization algorithms to solve the objective function. It solves the random fluctuation problem of photovoltaic and wind power output. This constitutes an independently controlled device. The system can meet both the demand for electricity and the grid’s needs. First, the micro-energy dispatching network’s controllability refers to the power grid’s overall single controllability. The control area of the entire network must be carried out in a relatively dense area. The system lacks flexibility. Secondly, the management of the micro-energy dispatching network pays more attention to autonomous administration in the control area, which requires a higher-level network management organization to realize the optimal operation of the access network. In addition, each micro-energy dispatching network requires a separate energy management unit.
In this way, the needs of large-scale distribution networks can be well met, thereby improving the overall utilization efficiency of the power market. This paper presents a dynamic energy optimization method for distributed generation energy. This paper focuses on the optimal closed-loop control performance in an optimization period in the future and introduces feedback correction. The method proposed in this paper can timely and effectively correct the deviation of optimal scheduling results caused by forecast errors and random factors. The manner in this paper can improve the precision of optimal control. The experimental results in this paper achieve the goal of “multi-level coordination, level-by-level refinement, feedback correction" and maximum consumption of intermittent distributed power. Finally, this paper uses examples and simulation methods to test the technology proposed in this paper.
2 Active and Reactive Power Coordination Scheduling Architecture Based on Distributed Power Sources
This paper divides the dynamic programming problem of multi-time-scale emotionally distributed generation energy into several epoch problems. The coordination relationship between each step is shown in Fig. 1.
Figure 1: Peak shaving optimization structure of distributed power energy based on distributed energy
1) Day-ahead optimal allocation: According to the forecast of day-ahead load and renewable energy, the optimal dispatch of active and reactive power of distributed generation energy is carried out. The optimal scheduling for the previous day is to determine the operating conditions and activities of the low-speed power units in the system for the next day. Low-speed dynamic devices mainly include power load regulators (OLTCs), compensation capacitors, and interruptible loads. Restricted by the production process and the device’s service life, the combination of power on-load voltage regulation and capacitor components leads to the micro-energy grid’s limited feeding rate and daily feeding quantity. Customers must be informed in advance to avoid unnecessary loss of power supply when implementing interruptible loads. The warning period is usually 2 to 1 working day. Such adjustable devices have a limited rate of adjustment. This method is not suitable for short-term scrolling and real-time feedback correction.
2) Daytime rolling optimal scheduling: Due to the extensive forecast time range, the instability factor of sustainable development and the considerable uncertainty of load forecasting, the forecast accuracy gradually decreases. The former scheduling scheme is difficult to adapt to the requirements of power balance. In the daily short-cycle rolling optimization, each cycle
3) Immediate correction: Although the fluctuation of renewable energy has a specific reduction in the load load of distributed power sources, it is still the optimal scheduling method for an open loop. In the smaller period
3 Global Optimal Control Strategy in Long Time Scale
Based on the load forecast of the following dispatch period and the power forecast of intermittent power, this paper uses the best optimal power algorithm to obtain the best allocation scheme of each controlled system. This method requires much information, a complicated algorithm, and much computation. It is suitable for overall optimal control of large volumes. This paper proposes an optimal design method based on dynamic programming for the overall operation of distributed power sources. The purpose is:
Each new renewable resource is considered to use Maximum Power Point Tracking (MPPT). Under the assumed conditions, the system can ignore the benefits of saving and reducing energy consumption, reducing costs. This indicator aims to consider and optimize the power supply demand of distributed power sources. In this way, the purpose of total power consumption of distributed power sources is achieved [7]. At the same time, because the grid price at peak time is higher than that at valley time, more power output is allocated to the feeder than at peak time. This method can improve the performance of the system. The optimal power flow constraints of distributed power sources are as follows: On the premise that the power supply lines cannot be transmitted in reverse, the conditions are:
4 Regional Autonomous Control Strategy on Short Time Scale
The external conditions and load requirements of distributed power sources are constantly changing. In this paper, a method of local autonomy is adopted for the dynamic characteristics of distributed power sources to realize the emotional response of dynamic attributes of active distributed power sources. At the same time, the system recognizes dynamic adjustment of dynamic load and dynamic characteristics of intermittent power supply. Assuming the optimal power flow optimized by the active distribution network, the target power
Through Eqs. (3) and (4), the regional autonomous control strategy in the short term can be expressed as:
From Eq. (6), it can be seen that after the autonomous control of each area is stabilized, if there is
It is assumed that the power disturbance
Figure 2: The principle of regional autonomous control
Figure 3: Short time scale active power optimization scheduling flow chart
Based on the IEEE3311 kV distribution network specification, this paper simulates and analyzes it. In the example, this paper proposes two kinds of dynamic power systems: the slow change of wind and photovoltaic; the sudden shift in wind and photovoltaic. Take 15 min as the maximum number of steps on a larger scale. This paper uses the original dual interior point method to optimize the power distribution and announces a new dynamic distribution system power distribution scheme after 1 h [12,13]. This article is based on the power output over the corresponding time interval [14,15]. At the same time, this paper uses M = N = 3 to carry out a rolling forecast to calculate the power of load, wind power and photovoltaic power generation within 15 min. In this paper, the continuous quadratic programming method is adopted, and the output power of the power system, such as load, wind and solar energy, is used as the input variable. In this paper, the gain of the dynamic output power of the system is obtained by the quadratic programming method. At the same time, the author adjusted the power of the long-term distributed power system so that the system’s short-term output power and long-term power output changed the most (Fig. 3) [16,17].
This article has re-planned the simulation example in Fig. 4. Fig. 4 shows the planned output value of active work on a long-term scale. The experimental condition is an economic dispatch model with the minimum operating power dispatch cost in the functional distribution network. Fig. 5 is a simulation diagram of short-term dynamic power output planning value. The model is short-time-scale optimal scheduling to minimize scheduling costs [18]. The short-time scale optimal scheduling takes the current actual active output of the system as the initial value of a new round of optimal rolling scheduling and forms a closed-loop control. This model overcomes the uncertainty of the system and wind power and photovoltaic. This way, the forecast value of the new round of active output is more realistic. Short-time-scale optimal scheduling takes the minimization of scheduling cost as the goal. This article has added this paragraph to the final draft. Fig. 6 shows the output value of each controllable distributed power source on a short time scale [19].
Figure 4: Load, wind and PV forecast data
Figure 5: The planned value of active power output of each distributed power generation on a long-term scale
Figure 6: Active power output of each distributed power source on the short time scale
The conventional open-loop optimization algorithm can optimally estimate the load, wind power and photovoltaic power generation in the optimal period at one time. The system allocates them optimally. Figs. 7a–7c compared the optimal sorting methods combining conventional open-loop and distributed energy [20–23]. A conclusion is drawn through simulation and analysis: this paper uses the optimal scheduling of distributed generation energy. Its power variation law is consistent with the power variation generated by the conventional open-loop optimal power flow algorithm, and the optimization effect is similar. However, different from the traditional open-loop optimal power flow allocation method, the rolling optimization scheme of power resources based on distributed generation takes the change of power output power as its parameter [24,25]. The system realizes the rolling optimization scheduling based on distributed power supply energy by feedback correction through real-time measurement data. Its running process is more stable. It can effectively resolve the fluctuations and uncertainties brought about by emergencies. The system ensures the stability of its output power, reduces its mechanical loss and increases the life of the distributed power supply.
Figure 7: Comparison of optimized scheduling results
This paper mainly studies the power load distribution problem in distributed power sources. It proposes a long-term optimal power flow based on the active power output of distributed power sources as the benchmark for the control scheme. This paper analyzes the power output of each controllable DG energy source using a multi-stage rolling optimization method. The example calculation shows that it is feasible to use this method to adjust the active power of distributed power sources. The dynamic energy optimization method of distributed power sources proposed in this paper can be extended and applied to distributed energy generation in microgrids and distribution planning and construction of electric vehicle charging piles. There are still contents that can be further deepened in the current research. Although the unit combination optimization model is discussed in this paper, factors such as the environment are not considered. Because the output of intermittent distributed power is greatly affected by natural elements, there is a significant prediction deviation in the existing prediction methods. At the same time, this paper fails to carry out comprehensive, coordinated planning for the distribution network, including distributed power generation and charging stations. This article only plans from the perspective of distributed power supply. The starting point of the article has certain limitations. The difference between the distributed power supply and the charging station planning scheme will also affect the construction and transformation of the distribution grid. One step can build a more accurate rolling forecast model for load, wind power and photovoltaic in model predictive control. This way, the rolling optimization process is based on more precise input information to obtain a more realistic active power output value.
In the future, it is necessary to deeply analyze the timing characteristics, frequency domain characteristics, structural characteristics and supply and demand characteristics of participating objects. At the same time, it is necessary to establish a comprehensive “source-network-load-storage" coordination and dispatching model. When doing research on load aggregation modeling, this paper needs to deeply study the coordination characteristics within the aggregator and establish a two-layer interactive aggregation model. The regional scale should be fully evaluated when choosing an active distribution network dispatch framework. At the same time, choose a more reasonable coordination scheduling architecture.
Funding Statement: The authors received no specific funding for this study.
Conflicts of Interest: The authors declare that they have no conflicts of interest to report regarding the present study.
References
1. Alatshan, M. S., Alhamrouni, I., Sutikno, T., Jusoh, A. (2020). Application of static synchronous compensator and energy storage system for power system stability enhancement. Bulletin of Electrical Engineering and Informatics, 9(6), 2222–2234. https://doi.org/10.11591/eei.v9i6.2319 [Google Scholar] [CrossRef]
2. Li, Y., Bai, X., Meng, J., Zheng, L. (2022). Multi-level refined power system operation mode analysis: A data-driven approach. IET Generation, Transmission & Distribution, 16(13), 2654–2680. https://doi.org/10.1049/gtd2.12481 [Google Scholar] [CrossRef]
3. Dong, Y., Shan, X., Yan, Y., Leng, X., Wang, Y. (2022). Architecture, key technologies and applications of load dispatching in China power grid. Journal of Modern Power Systems and Clean Energy, 10(2), 316–327. https://doi.org/10.35833/MPCE.2021.000685 [Google Scholar] [CrossRef]
4. Balsamo, F., de Falco, P., Mottola, F., Pagano, M. (2020). Power flow approach for modeling shipboard power system in presence of energy storage and energy management systems. IEEE Transactions on Energy Conversion, 35(4), 1944–1953. https://doi.org/10.1109/TEC.2020.2997307 [Google Scholar] [CrossRef]
5. Zhang, H., Ma, S., Ding, T., Lin, Y., Shahidehpour, M. (2020). Multi-stage multi-zone defender-attacker-defender model for optimal resilience strategy with distribution line hardening and energy storage system deployment. IEEE Transactions on Smart Grid, 12(2), 1194–1205. https://doi.org/10.1109/TSG.2020.3027767 [Google Scholar] [CrossRef]
6. Pan, E., Liu, S., Liu, J., Qi, Q., Guo, Z. (2020). The state grid corporation of China’s practice and outlook for promoting new energy development. Energy Conversion and Economics, 1(2), 71–80. https://doi.org/10.1049/enc2.12007 [Google Scholar] [CrossRef]
7. Anees, H. M., Kazmi, S. A. A., Naqvi, M., Naqvi, S. R., Dastgeer, F. et al. (2021). A mathematical model-based approach for DC multi-microgrid performance evaluations considering intermittent distributed energy resources, energy storage, multiple load classes, and system components variations. Energy Science & Engineering, 9(10), 1919–1934. https://doi.org/10.1002/ese3.901 [Google Scholar] [CrossRef]
8. Dai, R., Esmaeilbeigi, R., Charkhgard, H. (2021). The utilization of shared energy storage in energy systems: A comprehensive review. IEEE Transactions on Smart Grid, 12(4), 3163–3174. https://doi.org/10.1109/TSG.2021.3061619 [Google Scholar] [CrossRef]
9. Zhu, H., Zhang, Y., Liu, F., Wei, W. (2020). Effect of excess hydrogen on hydrogen fueled internal combustion engine under full load. International Journal of Hydrogen Energy, 45(39), 20419–20425. https://doi.org/10.1016/j.ijhydene.2019.12.022 [Google Scholar] [CrossRef]
10. Pla, B., de la Morena, J., Bares, P., Jiménez, I. (2020). Cycle-to-cycle combustion variability modelling in spark ignited engines for control purposes. International Journal of Engine Research, 21(8), 1398–1411. https://doi.org/10.1177/1468087419885754 [Google Scholar] [CrossRef]
11. Wang, X., Sun, B. G., Luo, Q. H. (2019). Energy and exergy analysis of a turbocharged hydrogen internal combustion engine. International Journal of Hydrogen Energy, 44(11), 5551–5563. https://doi.org/10.1016/j.ijhydene.2018.10.047 [Google Scholar] [CrossRef]
12. Malé, Q., Staffelbach, G., Vermorel, O., Misdariis, A., Ravet, F. et al. (2019). Large eddy simulation of pre-chamber ignition in an internal combustion engine. Flow, Turbulence and Combustion, 103(2), 465–483. https://doi.org/10.1007/s10494-019-00026-y [Google Scholar] [CrossRef]
13. Li, Y., Gao, W., Zhang, P., Ye, Y., Wei, Z. (2019). Effects study of injection strategies on hydrogen-air formation and performance of hydrogen direct injection internal combustion engine. International Journal of Hydrogen Energy, 44(47), 26000–26011. https://doi.org/10.1016/j.ijhydene.2019.08.055 [Google Scholar] [CrossRef]
14. Gainey, B., Longtin, J. P., Lawler, B. (2019). A guide to uncertainty quantification for experimental engine research and heat release analysis. SAE International Journal of Engines, 12(5), 509–290. https://doi.org/10.4271/03-12-05-0033 [Google Scholar] [CrossRef]
15. Thawko, A., Yadav, H., Eyal, A., Shapiro, M., Tartakovsky, L. (2019). Particle emissions of direct injection internal combustion engine fed with a hydrogen-rich reformate. International Journal of Hydrogen Energy, 44(52), 28342–28356. https://doi.org/10.1016/j.ijhydene.2019.09.062 [Google Scholar] [CrossRef]
16. Wang, L., Dubey, A., Gebremedhin, A. H., Srivastava, A. K., Schulz, N. (2022). MPC-based decentralized voltage control in power distribution systems with EV and PV coordination. IEEE Transactions on Smart Grid, 13(4), 2908–2919. https://doi.org/10.1109/TSG.2022.3156115 [Google Scholar] [CrossRef]
17. Li, B., Chen, Y., Wei, W., Wang, Z., Mei, S. (2022). Online coordination of LNG tube trailer dispatch and resilience restoration of integrated power-gas distribution systems. IEEE Transactions on Smart Grid, 13(3), 1938–1951. https://doi.org/10.1109/TSG.2022.3141548 [Google Scholar] [CrossRef]
18. Pereira, K., Pereira, B. R., Contreras, J., Mantovani, J. R. (2018). A multiobjective optimization technique to develop protection systems of distribution networks with distributed generation. IEEE Transactions on Power Systems, 33(6), 7064–7075. https://doi.org/10.1109/TPWRS.2018.2842648 [Google Scholar] [CrossRef]
19. Lei, S., Chen, C., Zhou, H., Hou, Y. (2018). Routing and scheduling of mobile power sources for distribution system resilience enhancement. IEEE Transactions on Smart Grid, 10(5), 5650–5662. https://doi.org/10.1109/TSG.2018.2889347 [Google Scholar] [CrossRef]
20. Wenzhi, S., Zhang, H., Tseng, M. L., Weipeng, Z., Xinyang, L. (2022). Hierarchical energy optimization management of active distribution network with multi-microgrid system. Journal of Industrial and Production Engineering, 39(3), 210–229. https://doi.org/10.1080/21681015.2021.1972478 [Google Scholar] [CrossRef]
21. Zhang, W., Xu, Y. (2018). Distributed optimal control for multiple microgrids in a distribution network. IEEE Transactions on Smart Grid, 10(4), 3765–3779. https://doi.org/10.1109/TSG.2018.2834921 [Google Scholar] [CrossRef]
22. Luo, F., Dong, Z. Y., Liang, G., Murata, J., Xu, Z. (2018). A distributed electricity trading system in active distribution networks based on multi-agent coalition and blockchain. IEEE Transactions on Power Systems, 34(5), 4097–4108. https://doi.org/10.1109/TPWRS.2018.2876612 [Google Scholar] [CrossRef]
23. Ehsan, A., Yang, Q. (2018). Coordinated investment planning of distributed multi-type stochastic generation and battery storage in active distribution networks. IEEE Transactions on Sustainable Energy, 10(4), 1813–1822. https://doi.org/10.1109/TSTE.2018.2873370 [Google Scholar] [CrossRef]
24. Yousaf, M., Jalilian, A., Muttaqi, K. M., Sutanto, D. (2022). An adaptive overcurrent protection scheme for dual-setting directional recloser and fuse coordination in unbalanced distribution networks with distributed generation. IEEE Transactions on Industry Applications, 58(2), 1831–1842. https://doi.org/10.1109/TIA.2022.3146095 [Google Scholar] [CrossRef]
25. Dashtpeyma, M., Ghodsi, R. (2022). Resilient electricity distribution network: Exploring research and managerial implications. Iranian Journal of Science and Technology, Transactions of Electrical Engineering, 46(1), 1–14. https://doi.org/10.1007/s40998-021-00453-6 [Google Scholar] [CrossRef]
Cite This Article
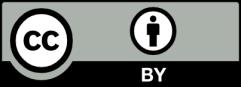