Open Access
ARTICLE
Robust Visual Tracking Models Designs Through Kernelized Correlation Filters
1 Fujian Provincial Academic Engineering Research Centre in Industrial Intellectual Techniques and Systems, College of Engineering, Huaqiao University, Quanzhou 362021, China
2 College of Mathematics and Computer Science, Quanzhou Normal University, Quanzhou 362000, China
3 Department of Computer Science and Information Engineering, National Quemoy University, No.1 University Rd, Kin-Ning Vallage Kinmen 892, Taiwan
* Corresponding Author: Hsuan-Ming Feng,
Intelligent Automation & Soft Computing 2020, 26(2), 313-322. https://doi.org/10.31209/2019.100000105
Abstract
To tackle the problem of illumination sensitive, scale variation, and occlusion in the Kernelized Correlation Filters (KCF) tracker, an improved robust tracking algorithm based on KCF is proposed. Firstly, the color attribute was introduced to represent the target, and the dimension of target features was reduced adaptively to obtain low-dimensional and illumination-insensitive target features with the locally linear embedding approach. Secondly, an effective appearance model updating strategy is designed, and then the appearance model can be adaptively updated according to the Peak-to-Sidelobe Ratio value. Finally, the low-dimensional color features and the HOG features are utilized to determine the target state to further improve the robustness of the tracker. The experimental results on OTB-2015 benchmark validate that the proposed tracker can effectively solve the illumination variation, scale variation, partial occlusion and deformation in the complex background.Keywords
Cite This Article
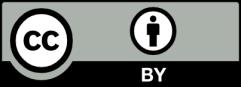