Open Access
ARTICLE
Automated Meter Reading Detection Using Inception with Single Shot Multi-Box Detector
Department of Computer Science, University of Balochistan, Quetta, Pakistan
* Corresponding Author: Arif Iqbal. Email:
Intelligent Automation & Soft Computing 2021, 27(2), 299-309. https://doi.org/10.32604/iasc.2021.014250
Received 09 September 2020; Accepted 14 December 2020; Issue published 18 January 2021
Abstract
Automated meter reading has recently been adopted by utility service providers for improving the reading and billing process. Images captured during meter reading are incorporated in consumer bills to prevent reporting false reading and ensure transparency. The availability of images captured during the meter reading process presents the potential of completely automating the meter reading process. This paper proposes a convolutional network-based multi-box model for the automatic meter reading. The proposed research leverages the inception model with a single shot detector to achieve high accuracy and efficiency compared to the existing state-of-the-art machine learning methods. We tested the multi-box detector with Mobile-Net and Faster Region-based Convolutional Neural Networks (R-CNN). The results depict that the proposed method not only outperforms the two baseline methods, but also requires less iterations (epochs) to train and rapidly improve precision with 96% accuracy. The dataset used for this research has been collected, preprocessed, and made publicly available to encourage further research and to serve as a baseline for the comparison.Keywords
Cite This Article
Citations
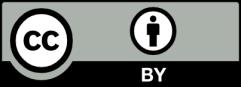