Open Access
ARTICLE
COVID-19 Diagnosis Using Transfer-Learning Techniques
1 College of Applied Computer Sciences, King Saud University, Riyadh, Saudi Arabia
2 Turabah University College, Taif University, Taif, Saudi Arabia
3 College of Computer and Information Science, King Saud University, Riyadh, Saudi Arabia
* Corresponding Author: Mohammed Faisal. Email:
(This article belongs to the Special Issue: Computational Intelligence for Internet of Medical Things and Big Data Analytics)
Intelligent Automation & Soft Computing 2021, 29(3), 649-667. https://doi.org/10.32604/iasc.2021.017898
Received 16 February 2021; Accepted 07 April 2021; Issue published 01 July 2021
Abstract
COVID-19 was first discovered in Wuhan, China, in December 2019 and has since spread worldwide. An automated and fast diagnosis system needs to be developed for early and effective COVID-19 diagnosis. Hence, we propose two- and three-classifier diagnosis systems for classifying COVID-19 cases using transfer-learning techniques. These systems can classify X-ray images into three categories: healthy, COVID-19, and pneumonia cases. We used two X-ray image datasets (DATASET-1 and DATASET-2) collected from state-of-the-art studies and train the systems using deep learning architectures, such as VGG-19, NASNet, and MobileNet2, on these datasets. According to the validation and testing results, our proposed diagnosis systems achieved excellent results with the VGG-19 architecture. The two-classifier diagnosis system achieved high sensitivity for COVID-19, with 99.5% and 100% on DATASET-1 and DATASET-2, respectively. The three-classifier diagnosis system achieves high sensitivity for COVID-19, with 98.4% and 100% on DATASET-1 and DATASET-2, respectively. The high sensitivity of these diagnostic systems for COVID-19 will significantly improve the speed and precision of COVID-19 diagnosis.Keywords
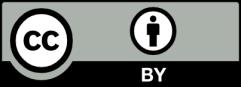