Open Access
ARTICLE
A Novel Classification Method with Cubic Spline Interpolation
1 Computer Science Department, Faculty of Science, University of Baghdad, Baghdad, Iraq
2 Faculty of Computer Science, Technology University, Baghdad, Iraq
3 Computer Science Department, Al-Turath University College, Baghdad, Iraq
* Corresponding Author: Husam Ali Abdulmohsin. Email:
(This article belongs to the Special Issue: Computational Intelligence for Internet of Medical Things and Big Data Analytics)
Intelligent Automation & Soft Computing 2022, 31(1), 339-355. https://doi.org/10.32604/iasc.2022.018045
Received 23 February 2021; Accepted 15 May 2021; Issue published 03 September 2021
Abstract
Classification is the last, and usually the most time-consuming step in recognition. Most recently proposed classification algorithms have adopted machine learning (ML) as the main classification approach, regardless of time consumption. This study proposes a statistical feature classification cubic spline interpolation (FC-CSI) algorithm to classify emotions in speech using a curve fitting technique. FC-CSI is utilized in a speech emotion recognition system (SERS). The idea is to sketch the cubic spline interpolation (CSI) for each audio file in a dataset and the mean cubic spline interpolations (MCSIs) representing each emotion in the dataset. CSI interpolation is generated by connecting the features extracted from each file in the feature extraction phase. The MCSI is generated by connecting the mean features of 70% of the files of each emotion in the dataset. Points on the CSI are considered the new generated features. To classify each audio file according to emotion, the Euclidian distance (ED) is found between each CSI and all MCSIs of all emotions in the dataset. Each audio file is classified according to the nearest MCSI to the CSI representing it. The three datasets used in this work are Ryerson Audio-Visual Database of Emotional Speech and Song (RAVDESS), Berlin (Emo-DB), and Surrey Audio-Visual Expressed Emotion (SAVEE). The proposed work shows fast classification and high accuracy of results. The classification accuracy, i.e., the proportion of samples assigned to the correct class, using FC-CSI without feature selection (FS), was 69.08%, 92.52%, and 89.1% with RAVDESS, Emo-DB, and SAVEE, respectively. The results of the proposed method were compared to those of a designed neural network called SER-NN. Comparisons were made with and without FS. FC-CSI outperformed SER-NN on Emo-DB and SAVEE, and underperformed on RAVDESS, without using an FS algorithm. It was noticed from experiments that FC-CSI operated faster than the same system utilizing SER-NN.Keywords
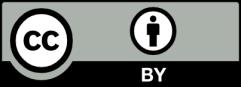