Open Access
ARTICLE
Dynamic Hyperparameter Allocation under Time Constraints for Automated Machine Learning
Korea Institute of Science and Technology Information (KISTI), Daejeon, 34140, Korea
* Corresponding Author: Sunil Ahn. Email:
Intelligent Automation & Soft Computing 2022, 31(1), 255-277. https://doi.org/10.32604/iasc.2022.018558
Received 12 March 2021; Accepted 15 April 2021; Issue published 03 September 2021
Abstract
Automated hyperparameter optimization (HPO) is a crucial and time-consuming part in the automatic generation of efficient machine learning models. Previous studies could be classified into two major categories in terms of reducing training overhead: (1) sampling a promising hyperparameter configuration and (2) pruning non-promising configurations. These adaptive sampling and resource scheduling are combined to reduce cost, increasing the number of evaluations done on more promising configurations to find the best model in a given time. That is, these strategies are preferred to identify the best-performing models at an early stage within a certain deadline. Although these time and resource constraints are significant for designing HPO strategies, previous studies only focused on parallel exploration efficiency using resource awareness. In this study, we propose a novel diversification strategy for HPO, which exploits the dynamic hyperparameter space allocation for a sampler according to the remaining time budget. We provide a simple yet effective method to accelerate the maturity of the sampler that is independent of the sampling algorithm. Compared to previous resource awareness solutions, our solution achieves better performance via both time and resource awareness. We demonstrate the performance gains of our solution on several well-known HPO benchmarks. Furthermore, we implement them to our high-performance computing AI convergence platform. Considering the different types of users, both a fully automated HPO service based on graphic processing unit (GUI) interfaces and HPO job management via python application programming interface (API) on the Jupyterlab are served on the platform, publicly.Keywords
Cite This Article
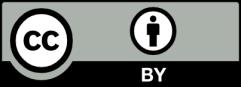