Open Access
ARTICLE
A Deep Learning to Distinguish COVID-19 from Others Pneumonia Cases
1 Laboratory of Advanced Technology and Intelligent Systems, National Engineering School, University of Sousse, Sousse, 4054, Tunisia
2 Laboratory of Computer engineering and systems, Faculty of Science and Technologies, Cadi Ayyad University, Marrakech, 40000, Morocco
* Corresponding Author: Sami Gazzah. Email:
Intelligent Automation & Soft Computing 2022, 31(2), 677-692. https://doi.org/10.32604/iasc.2022.019360
Received 11 April 2021; Accepted 18 May 2021; Issue published 22 September 2021
Abstract
A new virus called SARS-CoV-2 appeared at the end of space 2019 in Wuhan, China. This virus immediately spread throughout the world due to its highly contagious nature. Moreover, SARS-CoV-2 has changed the way of our life and has caused a huge economic and public health disaster. Therefore, it is urgent to identify positive cases as soon as possible and treat them as isolated. Automatic detection of viruses using computer vision and machine learning will be a valuable contribution to detecting and limiting the spread of this epidemic. The delay introduction of X-ray technology as diagnostic tool limited our ability to distinguish COVID-19 from other pneumonia cases. These images can be feed into a machine learning system that can be trained to detect lung infections, which can arise in acute pneumonia. However, some efforts attempt to detect SARS-CoV-2 using binary data and such combinations, which may leading to confusion. Indeed, in this case, the classifier’s training minimizes the intra-class similarity between pneumonia in COVID-19 and typical pneumonia. Due to the fact that in addition to SARS-CoV-2 pneumonia, there are two other common types: viral and bacterial pneumonia. They all appear in similar shapes, thus making them difficult to distinguish. This research proposed a deep multi-layered classification of pneumonia based on a neural network using chest X-ray images. The experiment was conducted using the combination of two images from an open-source dataset collected by Cohen GP and Kagel. The data consists of 4 categories: normal pneumonia, bacterial pneumonia, viral pneumonia, and COVID19, for 2,433 images. We considered two architectures: Xception and ResNet50. In addition, a comparison was made between the two models. The pre-trained Xception model in 20 epochs provided a classification accuracy of 86%.Keywords
Cite This Article
Citations
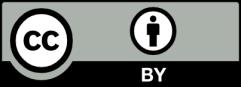