Open Access
ARTICLE
Video Surveillance-Based Urban Flood Monitoring System Using a Convolutional Neural Network
1 Department of Computer Science, King Khalid University-Sarat Abidha Campus, Abha, Saudi Arabia
2 Department of Computer Engineering, King Khalid University, Abha, Saudi Arabia
* Corresponding Author: R. Dhaya. Email:
Intelligent Automation & Soft Computing 2022, 32(1), 183-192. https://doi.org/10.32604/iasc.2022.021538
Received 06 July 2021; Accepted 07 August 2021; Issue published 26 October 2021
Abstract
The high prevalence of urban flooding in the world is increasing rapidly with the rise in extreme weather events. Consequently, this research uses an Automatic Flood Monitoring System (ARMS) through a video surveillance camera. Initially, videos are collected from a surveillance camera and converted into video frames. After converting the video frames, the water level can be identified by using a Histogram of oriented Gradient (HoG), which is used to remove the functionality. Completing the extracted features, the frames are enhanced by using a median filter to remove the unwanted noise from the image. The next step is water level classifiers using a Convolutional Neural Network (CNN), which is utilized to classify the water level in the images. The performance analysis of the method is analyzed by various parameters. The accuracy of the proposed method is 11% higher than that of the k-Nearest Neighbors (KNN) classifiers and 5% higher than that of the ANN classifiers, and the processing time is 7% less than that of the KNN classifiers and 4% less than that of the Artificial Neural Network (ANN) classifiers.Keywords
Cite This Article
Citations
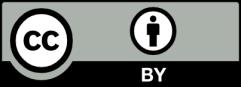