Open Access
ARTICLE
Machine Learning Approach for Improvement in Kitsune NID
1Department of Computer, College of Sciences and Arts in Al-Rass, Qassim University, Al-Rass, Saudi Arabia
2 Shaheed Zulfikar Ali Bhutto Institute of Science and Technology, Karachi, Pakistan
* Corresponding Author: Syed Sajjad Hussain Rizvi. Email:
(This article belongs to the Special Issue: Humans and Cyber Security Behaviour)
Intelligent Automation & Soft Computing 2022, 32(2), 827-840. https://doi.org/10.32604/iasc.2022.021879
Received 18 July 2021; Accepted 26 August 2021; Issue published 17 November 2021
Abstract
Network intrusion detection is the pressing need of every communication network. Many network intrusion detection systems (NIDS) have been proposed in the literature to cater to this need. In recent literature, plug-and-play NIDS, Kitsune, was proposed in 2018 and greatly appreciated in the literature. The Kitsune datasets were divided into 70% training set and 30% testing set for machine learning algorithms. Our previous study referred that the variants of the Tree algorithms such as Simple Tree, Medium Tree, Coarse Tree, RUS Boosted, and Bagged Tree have reported similar effectiveness but with slight variation inefficiency. To further extend this investigation, we have explored the performance of variants of above said Tree algorithms on other datasets provided by Kitsune, such as Active Wiretap, ARP MitM, Fuzzing, OS Scan, SSDP Flood, SYN DoS, SSL renegotiation, Mirai, and Video Injection. This investigation ascertains the likely performance of above said tree algorithm variants. After a deep and rigorous analysis, the Fine Tree is highly recommended for the improved version of the Kitsune Tool.Keywords
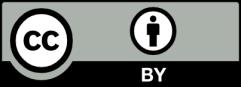