Open Access
ARTICLE
Synovial Sarcoma Classification Technique Using Support Vector Machine and Structure Features
1 Department of Electronics and Communication, K.L.N. College of Engineering, Madurai, 630612, India
2 School of Computer Science and Engineering, Vellore Institute of Technology (VIT), Chennai, 600127, India
3 School of Computer Science and Engineering, Centre for Cyber Physical Systems, Vellore Institute of Technology (VIT), Chennai, 600127, India
4 Department of Computer Science and Engineering, Gulzar Group of Institutions, Khanna, 141401, India
5 Department of Information Technology, Sikkim Manipal Institute of Technology, Sikkim Manipal University, Sikkim, 737136, India
* Corresponding Author: N. Janakiraman. Email:
(This article belongs to the Special Issue: Healthcare Intelligence in Cancer Prognosis and Prediction)
Intelligent Automation & Soft Computing 2022, 32(2), 1241-1259. https://doi.org/10.32604/iasc.2022.022573
Received 11 August 2021; Accepted 13 September 2021; Issue published 17 November 2021
Abstract
Digital clinical histopathology technique is used for accurately diagnosing cancer cells and achieving optimal results using Internet of Things (IoT) and blockchain technology. The cell pattern of Synovial Sarcoma (SS) cancer images always appeared as spindle shaped cell (SSC) structures. Identifying the SSC and its prognostic indicator are very crucial problems for computer aided diagnosis, especially in healthcare industry applications. A constructive framework has been proposed for the classification of SSC feature components using Support Vector Machine (SVM) with the assistance of relevant Support Vectors (SVs). This framework used the SS images, and it has been transformed into frequency sub-bands using Discrete Wavelet Transform (DWT). The sub-band wavelet coefficients of SSC and other Structure Features (SF) are extracted using Principle Component Analysis (PCA), Linear Discriminant Analysis (LDA), and Quadratic Discriminant Analysis (QDA) techniques. Here, the maximum and minimum margin between hyperplane values of the kernel parameters are adjusted periodically as a result of storing the SF values of the SVs in the IoT devices. The performance characteristics of internal cross-validation and its statistical properties are evaluated by cross-entropy measures and compared by nonparametric Mann-Whitney U test. The significant differences in classification performance between the techniques are analyzed using the receiver operating characteristics (ROC) curve. The combination of QDA + SVM technique will be required for intelligent cancer diagnosis in the future, and it gives reduced statistic parameter feature set with greater classification accuracy. The IoT network based QDA + SVM classification technique has led to the improvement of SS cancer prognosis in medical industry applications using blockchain technology.Keywords
Cite This Article
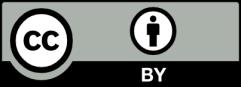