Open Access
ARTICLE
An Enhanced Re-Ranking Model for Person Re-Identification
Department of Computer Science and Engineering, School of Computing, SRM Institute of Science and Technology, Potheri, Kattankulathur, 603203, Tamil Nadu, India
* Corresponding Author: Jayavarthini Chockalingam. Email:
Intelligent Automation & Soft Computing 2022, 33(2), 697-710. https://doi.org/10.32604/iasc.2022.024142
Received 06 October 2021; Accepted 22 November 2021; Issue published 08 February 2022
Abstract
Presently, Person Re-IDentification (PRe-ID) acts as a vital part of real time video surveillance to ensure the rising need for public safety. Resolving the PRe-ID problem includes the process of matching observations of persons among distinct camera views. Earlier models consider PRe-ID as a unique object retrieval issue and determine the retrieval results mainly based on the unidirectional matching among the probe and gallery images. But the accurate matching might not be present in the top-k ranking results owing to the appearance modifications caused by the difference in illumination, pose, viewpoint, and occlusion. For addressing these issues, a new Hyper-parameter Optimized Deep Learning (DL) approach with Expanded Neighborhood Distance Reranking (HPO-DLDN) model is proposed for PRe-ID. The proposed HPO-DLDN involves different processes for PRe-ID, such as feature extraction, similarity measurement, and feature re-ranking. The HPO-DLDN model uses a Adam optimizer with Densely Connected Convolutional Networks (DenseNet169) model as a feature extractor. Additionally, Euclidean distance-based similarity measurement is employed to determine the resemblance between the probe and gallery images. Finally, the HPO-DLDN model incorporated ENDR model to re-rank the outcome of the person-reidentification along with Mahalanobis distance. An extensive experimental analysis is carried out on CUHK01 benchmark dataset and the obtained results verified the effective performance of the HPO-DLDN model in different aspects.Keywords
Cite This Article
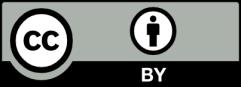