Open Access
ARTICLE
Image Masking and Enhancement System for Melanoma Early Stage Detection
Electrical and Electronics Eng. Dep., Kirikkale University, Kirikkale, 71450, Turkey
* Corresponding Author: Fikret Yalcinkaya. Email:
Intelligent Automation & Soft Computing 2022, 33(3), 1961-1977. https://doi.org/10.32604/iasc.2022.024961
Received 06 November 2021; Accepted 27 December 2021; Issue published 24 March 2022
Abstract
Early stage melanoma detection (ESMD) is crucial as late detection kills. Computer aided diagnosis systems (CADS) integrated with high level algorithms are major tools capable of ESMD with high degree of accuracy, specificity, and sensitivity. CADS use the image and the information within the pixels of the image. Pixels’ characteristics and orientations determine the colour and shapes of the images as the pixels and associated environment are closely interrelated with the lesion. CADS integrated with Convolutional Neural Networks (CNN) specifically play a major role for ESMD with high degree of accuracy. The proposed system has two steps to produce high degree of accuracy; the first is the Fuzzy-Logic (FL) based maskProd and pixel Enhancement vectors and the second is integration with deep CNN algorithms. The vectors, maskProd and pixel Enhancement, are based on lesion masking and image enhancement via fuzzy logic as hSvMask production has three inputs; sChannel, entropyFilter and distanceVector. The originality of this paper is based on the regional enhancement of each pixel of the image by fuzzy-logic. To change the brightness of an image by the conventional methods (CM), it is required to multiply the value of all and each pixels of the image by the same constant or weight value in order to generate new values of the pixels. But in the proposed method, FL determines a special and specific constant or weight value for every single pixel of the image; called a variable weight value. Secondly via conventional or classical methods of image processing, mask production is realized based on the threshold value predetermined, and this threshold value decides whether the image lesion is melanoma or not. CM use constant weight values but in the method suggested, FL determines a specific variable weight value for every single pixel of the image. Secondly CMs realize the mask production using predetermined threshold values, but proposed method suggests a new way capable of detecting pixels in the lesion-free regions, to do that, a system using FL was developed for each and every single pixel via the data obtained from three sources: the s-channel of the HSV (Hue, Saturation and Value) colour space, distance vector, and image entropy vector. The proposed method and its integration with algorithms; such as AlexNet, GoogLeNet, Resnet18, VGG-16, VGG-19, Inception-V3, ShuffleNet and Xception, detected the melanoma with an accuracy of 86.95, 85.60, 86.45, 86.70, 85.47, 86.76, 86.45 and 85.30 percentages, respectively.Keywords
Cite This Article
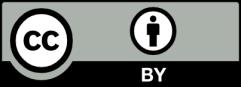