Open Access
ARTICLE
Bayesian Feed Forward Neural Network-Based Efficient Anomaly Detection from Surveillance Videos
Department of Computer Science and Engineering, M. Kumarasamy College of Engineering, Tamil Nadu, India
* Corresponding Author: M. Murugesan. Email:
Intelligent Automation & Soft Computing 2022, 34(1), 389-405. https://doi.org/10.32604/iasc.2022.024641
Received 25 October 2021; Accepted 02 January 2022; Issue published 15 April 2022
Abstract
Automatic anomaly activity detection is difficult in video surveillance applications due to variations in size, type, shape, and objects’ location. The traditional anomaly detection and classification methods may affect the overall segmentation accuracy. It requires the working groups to judge their constant attention if the captured activities are anomalous or suspicious. Therefore, this defect creates the need to automate this process with high accuracy. In addition to being extraordinary or questionable, the display does not contain the necessary recording frame and activity standard to help the quick judgment of the parts’ specialized action. Therefore, to reduce the wastage of time and labor, this work utilizes a Bayesian Feed-Forward Neural Network (BFFN) algorithm to automate the anomaly Recognition System. It aims to detect irregularities, regular signs of aggression, and violence automatically, rather than the conventional methods. This work uses Bayesian Feed Forward Neural Networks to identify and classify frames with high activity levels. From there, the proposed system can issue detection alerts for threats indicating suspicious activity at a particular moment. The simulation results show that the proposed BFFN based anomaly detection responds better than existing methods. The sensitivity, specificity, accuracy, and F1-score of the proposed system are 98.69%, 97.01%, 99.65%, and 0.98%.Keywords
Cite This Article
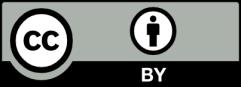