Open Access
ARTICLE
Classification of Multi-Frame Human Motion Using CNN-based Skeleton Extraction
1 Contents Convergence Software Research Institute, Kyonggi University, Suwon-si, 16227, Korea
2 Division of AI Computer Science and Engineering, Kyonggi University, Suwon-si, 16227, Korea
* Corresponding Author: Kyungyong Chung. Email:
Intelligent Automation & Soft Computing 2022, 34(1), 1-13. https://doi.org/10.32604/iasc.2022.024890
Received 03 November 2021; Accepted 23 December 2021; Issue published 15 April 2022
Abstract
Human pose estimation has been a major concern in the field of computer vision. The existing method for recognizing human motion based on two-dimensional (2D) images showed a low recognition rate owing to motion depth, interference between objects, and overlapping problems. A convolutional neural network (CNN) based algorithm recently showed improved results in the field of human skeleton detection. In this study, we have combined human skeleton detection and deep neural network (DNN) to classify the motion of the human body. We used the visual geometry group network (VGGNet) CNN for human skeleton detection, and the generated skeleton coordinates were composed of three-dimensional (3D) vectors according to time changes. Based on these data, we used a DNN to identify and classify human motions that were most similar to the existing learned motion data. We applied the generated model to the data set that could occur in general closed circuit television (CCTV) to check the accuracy. The configured learning model showed effective results even with two-dimensional continuous image data composed of red, green, blue (RGB).Keywords
Cite This Article
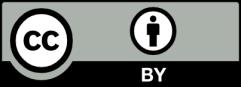