Open Access
ARTICLE
IoT Based Disease Prediction Using Mapreduce and LSQN3 Techniques
1 Department of Computer Science and Engineering, Dhanalakshmi Srinivasan Engineering College, Perambalur, 621212, India
2 Department of Computer Science and Engineering, SRM Institute of Science and Technology, Ramapuram, 600089, India
3 Directorate of Distance Education, Alagappa University, Karaikudi, 630003, India
4 Department of Electrical and Electronics Engineering, Sathyabama Institute of Science and Technology, Chennai, 600119, India
5 Department of Information Technology, Sona College of Technology, Salem, 636005, India
6 Department of Computer Science and Engineering, VNR Vignana Jyothi Institute of Engineering and Technology, Hyderabad, 500090, India
7 Department of Computer Science, KIET Group of Institutions, Delhi NCR, Ghaziabad, 201206, India
* Corresponding Author: R. Gopi. Email:
Intelligent Automation & Soft Computing 2022, 34(2), 1215-1230. https://doi.org/10.32604/iasc.2022.025792
Received 05 December 2021; Accepted 19 January 2022; Issue published 03 May 2022
Abstract
In this modern era, the transformation of conventional objects into smart ones via internet vitality, data management, together with many more are the main aim of the Internet of Things (IoT) centered Big Data (BD) analysis. In the past few years, significant augmentation in the IoT-centered Healthcare (HC) monitoring can be seen. Nevertheless, the merging of health-specific parameters along with IoT-centric Health Monitoring (HM) systems with BD handling ability is turned out to be a complicated research scope. With the aid of Map-Reduce and LSQN3 techniques, this paper proposed IoT devices in Wireless Sensors Networks (WSN) centered BD Mining (BDM) approach. Initially, the heart disease prediction dataset is acquired from publicly available sources in the proposed approach. Following that, the dataset is mitigated by reducing redundant data using Map-Reduce and making it useful for the upcoming examination. During the mapping step, the Linear Log induced K-Means Algorithm (LL-KMA) clustering algorithm is used. The LF-CSO technique is used in the reduction phase to select the optimal Cluster Centroids (CC). The features are extracted from the reduced data. After that, utilizing the Pearson Correlation Coefficient based Generalized Discriminant Analysis (PCC-GDA), the extracted features’ dimensionality is mitigated. Subsequently, the features being reduced are neumaralised for classification purposes. Lastly, to classify the disease, the Log Sigmoid activation based Quasi-Newton Neural network (LSQN3) classifier is employed. The proposed method is contrasted with the existing methodologies to assess the performance. The experiential outcomes displayed that the proposed work is highly efficient than the other methodologies.Keywords
Cite This Article
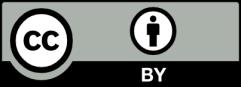