Open Access
ARTICLE
Deep Learning Based Residual Network Features for Telugu Printed Character Recognition
1 Department of Computer Science and Engineering, FEAT, Annamalai University, Chidambaram, 608002, Tamilnadu, India
2 Department of Networking and Communications, School of Computing, SRM Institute of Science &Technology, Kattankulathur, 603203, Tamilnadu, India
* Corresponding Author: Vijaya Krishna Sonthi. Email:
Intelligent Automation & Soft Computing 2022, 34(3), 1725-1736. https://doi.org/10.32604/iasc.2022.026940
Received 07 January 2022; Accepted 10 February 2022; Issue published 25 May 2022
Abstract
In India, Telugu is one of the official languages and it is a native language in the Andhra Pradesh and Telangana states. Although research on Telugu optical character recognition (OCR) began in the early 1970s, it is still necessary to develop effective printed character recognition for the Telugu language. OCR is a technique that aids machines in identifying text. The main intention in the classifier design of the OCR systems is supervised learning where the training process takes place on the labeled dataset with numerous characters. The existing OCR makes use of patterns and correlations to differentiate words from other components. The development of deep learning (DL) techniques is useful for effective printed character recognition. In this context, this paper introduces a novel DL based residual network model for printed Telugu character recognition (DLRN-TCR). The presented model involves four processes such as preprocessing, feature extraction, classification, and parameter tuning. Primarily, the images of various sizes are normalized to 64x64 by the use of the bilinear interpolation technique and scaled to the 0, 1 range. Next, residual network-152 (ResNet 152) model-based feature extraction and then Gaussian native Bayes (GNB) based classification process is performed. The performance of the proposed model has been validated against a benchmark Telugu dataset. The experimental outcome stated the superiority of the proposed model over the state of art methods with a superior accuracy of 98.12%.Keywords
Cite This Article
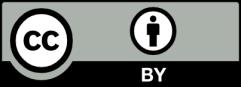