Open Access
ARTICLE
Game Theory-Based Dynamic Weighted Ensemble for Retinal Disease Classification
Department of CSE, CEG, Anna University, Chennai, 600025, India
* Corresponding Author: Kanupriya Mittal. Email:
Intelligent Automation & Soft Computing 2023, 35(2), 1907-1921. https://doi.org/10.32604/iasc.2023.029037
Received 23 February 2022; Accepted 01 April 2022; Issue published 19 July 2022
Abstract
An automated retinal disease detection system has long been in existence and it provides a safe, no-contact and cost-effective solution for detecting this disease. This paper presents a game theory-based dynamic weighted ensemble of a feature extraction-based machine learning model and a deep transfer learning model for automatic retinal disease detection. The feature extraction-based machine learning model uses Gaussian kernel-based fuzzy rough sets for reduction of features, and XGBoost classifier for the classification. The transfer learning model uses VGG16 or ResNet50 or Inception-ResNet-v2. A novel ensemble classifier based on the game theory approach is proposed for the fusion of the outputs of the transfer learning model and the XGBoost classifier model. The ensemble approach significantly improves the accuracy of retinal disease prediction and results in an excellent performance when compared to the individual deep learning and feature-based models.Keywords
Cite This Article
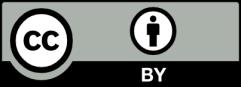