Open Access
ARTICLE
Design and Development of Low-cost Wearable Electroencephalograms (EEG) Headset
1 Department of Electrical Engineering, Sukkur IBA University, 65200, Sukkur, Pakistan
2 Department of Electronic Engineering, Quaid-e-Awam University of Engineering, Science and Technology, Larkana, 77150, Pakistan
3 Faculty of Engineering and AlShrouk Trading Company, Najran University, Najran, 61441, Saudi Arabia
4 Department of Medical Rehabilitation Sciences-Physiotherapy Program, College of Applied Medical Sciences, Najran University, Najran, 61441, Saudi Arabia
5 Computer Engineering Department, Yarmouk University, Irbid, 21163, Jordan
6 Pre-Clinical Research Unit, King Fahd Medical Research Center, King Abdulaziz University, Jeddah, 21589, Saudi Arabia
7 Department of Medical Laboratory Technology, Faculty of Applied Medical Sciences, King Abdulaziz University, Jeddah, 21589, Saudi Arabia
8 Department of Internal Medicine, Medical College, Najran University, Najran, 61441, Saudi Arabia
9 Electrical Engineering Department, College of Engineering, Najran University Saudi Arabia, Najran, 61441, Saudi Arabia
10 Novel Global Community Educational Foundation, Hebersham, NSW 2770, Australia, AFNP Med Austria, Wien, 2770, Austria
* Corresponding Author: Toufique Ahmed Soomro. Email:
Intelligent Automation & Soft Computing 2023, 35(3), 2821-2835. https://doi.org/10.32604/iasc.2023.026279
Received 21 December 2021; Accepted 20 March 2022; Issue published 17 August 2022
Abstract
Electroencephalogram (EEG) is a method of capturing the electrophysiological signal of the brain. An EEG headset is a wearable device that records electrophysiological data from the brain. This paper presents the design and fabrication of a customized low-cost Electroencephalogram (EEG) headset based on the open-source OpenBCI Ultracortex Mark IV system. The electrode placement locations are modified under a 10–20 standard system. The fabricated headset is then compared to commercially available headsets based on the following parameters: affordability, accessibility, noise, signal quality, and cost. First, the data is recorded from 20 subjects who used the EEG Headset, and signals were recorded. Secondly, the participants marked the accuracy, set up time, participant comfort, and participant perceived ease of set-up on a scale of 1 to 7 (7 being excellent). Thirdly, the self-designed EEG headband is used by 5 participants for slide changing. The raw EEG signal is decomposed into a series of band signals using discrete wavelet transform (DWT). Lastly, these findings have been compared to previously reported studies. We concluded that when used for slide-changing control, our self-designed EEG headband had an accuracy of 82.0 percent. We also concluded from the results that our headset performed well on the cost-effectiveness scale, had a reduced setup time of 2 ± 0.5 min (the shortest among all being compared), and demonstrated greater ease of use.Keywords
The human brain consists of billions of neurons that communicate with one another through electrical activity [1]. This brain activity can be recorded using electroencephalography (EEG), a technique that detects the potential difference between different points on the human brain. EEG measurements necessitate the placement of EEG electrodes in the 10–20 standard system, as illustrated in Fig. 1. Typically, this placement is done manually by an expert or, more commonly, with flexible electrode caps that stretch over the head of users.
Figure 1: 10–20 electrode placement standard. Even numbers (2–10): right hemisphere, numbers increase from midline z; Odd numbers (1–9): left hemisphere, numbers increase from midline z
Brain-computer interfaces (BCIs) based on electroencephalograms (EEGs) have been extensively researched in recent decades to assist challenged individuals in communicating with the outside world [2]. Various BCI applications, such as mental spellers [3], patient-assistant systems [4], neuro-rehabilitation [5], and external device control [6] have been developed for these people, showing the potential of EEG-based BCIs as practical assistance tools. The EEG headset converts data into packets and transmits them to a Personal Computer (PC) over a wireless medium (Bluetooth). The Brain-Computer Interface (BCI) system allows communication between the headset and the PC [7].
Recently, with the increasing public interest in BCI technology, BCIs have been developed for healthy people. Especially, the rapid advancement in the system-on-a-chip (SoC) technology has facilitated the release of wearable EEG devices that can be used for commercial BCI applications [8]. For instance, InteraXon Inc. launched MuseTM, a headband-type wearable EEG device that helps in meditation. Other examples include EmotiveEPOC+ (Emotive Inc.), Mindwave Mobile 2 (Neurosky Inc.), and BIOSEMI (Brain Products Inc.). While these wearable devices have limitations in terms of signal quality [9], yet represent advantages over the conventional EEG devices in that they are more economical, light in weight, portable, and easy to use [10]. These wearable EEG devices have been employed for passive BCI (pBCI) applications. This rapidly growing category of EEG devices aims at decoding the users’ mental state, such as emotions, sleep patterns, reasoning, relaxation, and attention [11].
The two types of wearable EEG devices currently in the market are Headband-type and headset-type devices. The EEG signals from the non-hair bearing region (i.e., forehead) are measured using headband-type devices. These devices are visually attractive, efficient, and easy to set up [12]. They monitor EEG signals from the forehead over the prefrontal cortex, which interprets different human mental states such as attention, emotion, and cognition [13]. On the other hand, EEG signals recorded from the frontal region of the brain are prone to contamination by artifacts, such as eye blinks, electrooculogram (EOG), and electromyogram (EMG) artifacts [14]. In addition to this, these devices cannot be used for certain applications because of a limited number of electrodes.
In contrast to headband type, the headset-type EEG devices can measure EEG signals from more brain locations: not only from the frontal region but also from other brain areas, comprising the temporal, parietal, and occipital cortices. EEG signals recorded from wider brain regions allow the BCI application to estimate the mental state of the user with higher accuracy [15]. This makes headset-type EEG devices suitable for a broader range of BCI applications than headband-type EEG devices. For example, global field synchronization—a measurement based on EEG signals collected from the entire brain—effectively predicts users’ emotional valence [16]. However, a substantial preparation procedure is generally needed for proper wearing of the devices because the electrodes must remain in close contact with the scalp surface in the hair-bearing areas [17].
Consumer-grade headsets have rapidly become the industry for research and academic use [18]. Many studies have compared the performance of signals received from different EEG sensors, but neither has evaluated the entire device, including expense, configuration time, ease of use, and quality [19]. BCIs inspire the integration and innovation of more and more devices. Because consumer-grade EEG systems are becoming more popular, stable, and accessible, they necessitate a more detailed comparison of the available headsets [20].
These devices are for particular use, such as sleeping pattern detection analysis [21], or meditations [22]. The ability to 3-D print with desired changes is one of the key features of our self-prepared headset and headband-type EEG devices. This feature not only lowers the total expense of the headset but also makes room for innovative improvements and commercialization potential. In this paper, we want to present a reliable evaluation of its functionality and price. Scientists have already conducted tests to evaluate the data of dry vs. wet EEG sensors [23], consumer-grade vs. clinical headset [24], a different number of electrodes [25], and signal quality [23,26]. The one thing that all of these experiments share in common is that they all have concentrated on showing the efficacy of some component of an EEG system, while our approach is comparing EEG systems as a whole.
The neocortex is the largest region of a mammal’s brain, covering nearly the whole brain except the cerebellum and accounting for roughly 76 percent of the total volume of the human brain [27]. Because the surface area of the brain and neurons have increased, the neocortex is also heavily wrinkled, making the brain considerably more efficient. Hemispheres are two symmetrical portions (e.g., right and left) of the cerebral cortex that have slightly distinct roles [28].
Brain-computer interfaces (BCIs) are specialized systems that read, evaluate, categorize and interpret brain waves, which are specific physiological signals [18]. The difference between a system that merely reads a physiological signal and a BCI is that the latter allows the user to interact with a computer or any other machine utilizing this signal by establishing a communication channel between the user’s brain and the device to operate. Traditional BCI applications mainly focus on enhancing disabled people’s interaction experiences [29]. People without disabilities, on the other hand, are prospective consumers of solutions that utilize brain signals for human-computer connection. Hence, brain waves are considered as the foundation for every BCI system [30], as all control signals extrapolate from these brain waves.
The leading neurological stimulus used for BCI systems is motor imagery (MI), in which subjects are encouraged to visualize executing a complex motor initiative, such as trying to move a foot or hand with no muscle strength. BCIs that focus on user MI has received greater attention in recent years, and MI-based electroencephalography (EEG) signal analysis has been the most widely used technology because of its comparatively low cost, ease of use, and reasonably harmless nature [31].
A BCI system are categorized according to a variety of factors, such as the nature of the employed device (invasive or noninvasive), the nature of the stimuli of the signal (exogenous or endogenous), the nature of the data processing methods (synchronous or asynchronous data processing), and how the control signal is applied (active, passive, or reactive) [32].
EEG is commonly utilized since it is a non-invasive technology that does not require a brain implant to obtain data from the user. Non-invasive devices have a lesser spatial resolution than invasive equipment [33], which is a drawback. The non-invasive EEG devices, however, poses no harm to the user because it simply detects impulses from the scalp [32,34] (i.e., the skin layer bordering the face and the neck).
First, we convert the raw signal into a series of bands using discrete decomposition techniques like wavelet decomposition (DWT) [35]. Different techniques used for noise removal available in the literature are independent component analysis (ICA) and principal component analysis (PCA) [36,37]. Data decomposition (DD) based techniques have recently gained importance in the pattern recognition of MI EEG signals [38]. Some notable DD based techniques available in the literature are empirical wavelet decomposition (EWT) and discrete wavelet decomposition (DWT) [39].
In this study, first, we have designed an EEG headband that can be used for recording the physiological activity of the prefrontal region of the brain. We have also developed an application of slide-changing control and measured its accuracy. Subsequently, we designed a general-purpose EEG headset that will assist paralyzed patients in employing the BCI application. Finally, general purpose electrode configurations were developed which can be used to maximize the overall performance of BCI applications. The following sections have to go through specific study procedures in depth.
The 16 electrodes on our prepared headset is placed in compliance with the 10–20 system’s electrode positioning configuration, which is as follows: As shown in Fig. 1.
Fig. 1 shows the 10–20 system [40], the international EEG electrode placement standard. This method was established to adopt standardized recording to ensure that any results of the study (research or clinical) could be assembled, replicated, compared, and analyzed in a consistent manner across the scientific community. Typically, this method labels the sensors based on their head position. The space between the nearby sensors is 20%/10% of the head size. This method of electrode placement reduces signal to noise ratio and increases the reliability of the signal [40].
The design specifications for the said headset-type prototype are given as follows: The prototype will be available in one size (i.e. large size for adults above 18), however, we can print it in different sizes as the 3D model requires only minimal changes to fit another size. It should cover the same electrode locations as covered by open source Ultracortix Mark IV (Fp1, Fpz, Fp2, Fc5, Fc3, Cz, Fc2, Fc4, T5, T6, P3, P4, T3, T4, O1, O2) having only 5 mm displacement from their original locations during movement, have an average positioning error of maximum 25 mm and can easily be placed on the head by the inexpert. The design was based on a previous work that presented a statistical shape model of the human scalp comprising 100 North-American subjects [20].
The design specifications for the headband-type prototype are given as follows: The prototype is a 3D print that is available in one size (i.e. for individuals above 18). It should cover the same electrode locations as covered by MUSE 2 headband (Fp1, Fpz, Fp2) having fixed positions with only 2–4 mm displacement from their original locations during movement and can easily be placed on the head by the inexpert.
The wide headset frame was 3-D printed as four different hemispheres, front-left, front-right, back-left, and back-right, following the instructions in the OpenBCI assembly guide [41]. Polylactic Acid (PLA) polymer is also used to print 25 insertion points for sensors as well as the microcontroller holder and reference electrode holder. The following parts were purchased: ear clips, comfort units, flat units, spikey units, screws, and ribbon cables. (refer to Fig. 5).
For this study, a 16-channel EEG Headset was designed (refer to Fig. 2), 3-D printed (refer to Fig. 3), and assembled (refer to Fig. 4).
Figure 2: The designed headset in Solidworks (a) Front-left (b) Front-right (c) Back_left (d) Back-right
Figure 3: The designed headset after 3-D printing (a) Front-left (b) Front-right (c) Back_left (d) Back-right
Figure 4: The printed headset (a) Without electrodes (b) With electrodes
Figure 5: The purchased electrodes (a) Spiky units (b) Comfort units (c) Flat units (d) Ear clips
3.3 Brain Computer Interface (BCI)
Fig. 7 illustrates the overall framework of the study including the Brain-Computer Interface system. The OpenBCI Biosensing Cyton Board [42] is used to collect EEG signals. The signals are then Bluetooth-transferred to the device (refer Fig. 6). Following headset configuration, the Open-BCI software was employed to check acquired EEG data quality. Using the OpenBCI master software, we analyzed the signal for noise, eye blinks, and Fast Fourier Transform (FFT) data.
Figure 6: (a) Biosensing Cyton Board (b) Bluetooth Module
Figure 7: The complete frame work of the study from headset 3D design to the EEG based classification algorithm to detect voluntary eye blinks
The headband kit is one of the most basic headsets, capable of acquiring EEG signals from five electrodes via the Cyton board. We collected data from Fp1, Fp2, and FpZ. The headset is created in Solidworks [43] (see Fig. 8a) and then printed in a 3-D printer (refer Fig. 8b). Fig. 8c depicts the headband with three electrodes at the previously mentioned locations.
Figure 8: Headband kit development (a) Design in Solidworks (b) Printing in 3-D printer at Fab-Lab (c) Headband kit with electrodes
A preliminary experiment to verify the design method was performed by 3 groups of undergraduate students (Final year Undergraduate in Electrical Engineering Department, Sukkur IBA University). The experiment aimed to see, if the suggested technique could meet the design criteria, and if the electrode placement, stability, and repeatability of the prototype generated using the 3D shape model were equivalent to those of a commercial headset. This was validated by comparing the three-dimensional locations of the fourteen electrode positions mentioned in section 2.1 to those of an Emotive EPOC+, as well as determining electrode position variation after movement and repeated set-up.
The sample consisted of 20 students (10 males and 10 females), all of whom were undergraduate and aged 18 to 25. There were no head deformations or head injuries in any of the participants. A consignment form has been signed by all the participants who took part in the experiment.
✽ Desktop computer running windows 10 and Master Software
✽ Emotive EPOC+
✽ Self-designed headset prototype
✽ Self-designed headband prototype
The experiment was split into two parts: one for headset-type EEG device and the other for headband-type EEG device. The participants were instructed to sit in front of the Personal Computer (PC) (see Fig. 9) where data were being recorded. The designed headset prototype was first placed on the user’s head as a guide for the optimal electrode placements. The sixteen essential electrode positions were calculated and compared with the 10–20 standard system. The reference electrodes were put on the earlobes, and the headset was positioned such that the frontal electrodes were roughly three fingers from the subject’s eyebrow, according to the directions in the electrode quick start handbook [44].
Figure 9: Subjects wearing headsets (a) Subject 1 wearing Emotive Epoc+ headset (b) Subject 1 wearing self-designed headset (c) Subject 2 wearing self-designed headset
Participants have given a questionnaire at the end of each session. We asked the participants to estimate how long it took to set up the headset in minutes (from the moment of head measurement until the decision of the experiment leader that signals were good). They then used a 7-point Likert scale to assess the setup speed (1 = extremely quick, 7 = very slow). On a 7-point Likert scale, the headset’s comfort (1 = very pleasant, 7 = very uncomfortable) and simplicity of setup (1 = very easy, 7 = very difficult) were assessed. Points 2–6 were left unlabeled in the middle (see Tab. 1). Finally, participants were given a mirror and had asked to rate their looks on a scale of 1 to 7 (with 7 being the best) while wearing the headset. Participants were also asked to rank each of the headsets, which they had all used by this point, in order of preference after the last session.
On the other hand, the experiment for validating the design of a headband-type EEG device is carried out by a group of 5 participants. The headband was put on the forehead of each participant sitting in front of a computer with a presentation being run on the computer. The experiment was run for 3 min for each participant. The participant was asked to blink their eyes voluntarily two times with approximately100 ms delay. When the slide successfully changed he told us ‘Yes’ and we noted it, when it failed to change or changed without eye blinking, he said ‘No’ and we noted it. However, the difficult part was to investigate whether it changes with threshold time or not. So, it contains some degree of tolerance. The slide-change with involuntary eye blinks had monitored by our team.
4 Results, Discussion and Future Work(s)
The experiment is conducted by collecting data from various subjects using our self-designed headset (refer to Fig. 9a) and Emotive Epoc+ headset (refer Figs. 9b and 9c). Tab. 1 summarizes the findings.
We analyzed the acquired data using third-party software MATLAB after analyzing the real-time data with OpenBCI master software. Fig. 10 shows a series of signal bands from the decomposition of raw EEG signals using discrete wavelet transformation (DWT).
Figure 10: Different signal bands derived from raw EEG signal
Peaks (high amplitude) with eye-blinks are visible in the raw EEG data. Because the Headsets were not prepared for data acquisition, the first part of the data has negative values. The raw EEG signal is decomposed into a series of band signals using discrete wavelet transform (DWT).
Tab. 1 shows that our designed headset-type EEG device has the shortest installation time (20.5 min) among all of four headsets being tested. The participants have expected more installation time as compared to actual installation time. The estimated values ranged from 2 to 20 min and 3 s, but the actual values ranged from 3 min and 5 s to 14 min and 4 s. The average score for ease of setup was 2, which is the highest of any headset compared. Self-designed customized open-source Mark IV headset performed well in terms of setup speed, where it tied with commercially available Emotive headset.
We utilized the cost-effectiveness ratios (CER) estimated by [45] and computed the cost, in euros, of the two headsets used in [46], then converted all prices from euros to USD for consistency. The prices is also converted from [47] to USD.
Researchers have used a method to regularize the unequal headset distinction, a separate metric for evaluating devices, price ratios (CERs). A CER plot of the three headsets has shown in Fig. 11. The self-designed EEG Headset-type device (marked as Mark IV) scored better on the effectiveness scale (82.90 percent) and fell into the cheaper range ($299) by considering the cost of all three devices (average = $7,907.29, maximum = $6,997). Based on these results, the self-designed EEG headset-type device offers the best value for money, with the 3rd cheapest cost and the best effectiveness scores in terms of ease of configuration, comfortability, and setup time. It costs around $12,701 less and has half the number of sensors as the Biosemi.
Figure 11: Price map for all devices based on offline classified data
As discussed in section 3.3.5 we have implemented a slide-changing application for paralyzed patients using our self-designed Headband-type EEG device. We have tested the application on 5 participants. All were healthy patients, as we haven’t found the paralyzed patients in our locality. Tab. 2 summarizes the experimental results.
Tab. 2 above results show that the accuracy for different persons was different, however, the overall accuracy was 82.0% which is satisfactory. We also noticed that once in all results for subject 3, the transition in the slide was difficult to identify as it has changed with eye-blink or not, so we discarded it from our investigation.
In a classical BCI paradigm, this study aimed to examine how a diverse group of participants would rate commercially available headsets in comparison with self-designed EEG devices in terms of their usefulness (efficacy, efficiency, and satisfaction) and visual design. We also looked at which headset participants preferred in the end, what factors seemed to influence it, and how these aspects interact. Finally, when the commercially available EEG headsets and the self-designed EEG devices (headband and headset) have been compared for several electrodes and electrode sites, we have also evaluated the classification accuracy of detection of voluntary eye-blinks. In this study, we have collected a dataset of 20 subjects, we can also increase the subjects up to 60 subjects [49].
Our designed and printed headset can be used to collect data from adults. Furthermore, we are also designing and developing the same type of headsets for children of various ages. In the future, this headset can be improved by using flexible material and customizing the electrode locations following the 10–20 standard system.
In the future, we will try to carry out a scientific investigation with blind subjects and compare their different EEG features with normal people. For improved results, more participants with increased time under different stimuli would be employed for the next analysis. Specifically, the post-COVID-19 era of facilitating long-distance online learning is another inspiration for future studies. Since classroom interaction between teachers and students is not possible with online teaching. The challenge of evaluating student knowledge in online learning is higher than classroom teaching. Hence, we intend to start looking deeply at the connection between EEG signals, focus, and learning.
The performance can be assessed by devising a specific experiment and comparing the accuracy with these parameters. These studies will aid in a more thorough understanding of the benefits and limitations of our prepared Mark-IV device. The price changes of the headset were not taken into account in this study. Change in costs will have a significant impact on the accessibility and cost-effectiveness ratio of EEGs, so they must be considered in future work.
According to the findings of current and previous studies, consumer-grade EEGs appear to be more accessible and less expensive than medical-grade EEGs. Using multiple parameters for comparison provides comprehensive information, but it can be misleading if electrodes are not placed in the similar locations for each headset. Although, the self-designed and fabricated Mark IV headset has performed well among the three headsets having these unbalanced circumstances. Moreover, the prepared headset can be employed for the BCIs and neurofeedback applications. However, this headset is not recommended for clinical applications.
Funding Statement: The Deanship of Scientific Research funded this work (DSR), King Abdulaziz University, Jeddah, Saudi Arabia, under grant no. (RG-18-130-43). The authors, therefore, acknowledge with thanks DSR technical and financial support. We also acknowledge the services of Sukkur IBA University Fab-Lab Research Associate Mr. Nadir Ali who helped us in printing the Headset.
Conflicts of Interest: The authors declare no conflicts of interest to report regarding the present study.
References
1. V. Bandara and A. Nanayakkara, “A low-cost, portable biopotential monitoring system to study electrical activities of the human brain and body,” European Journal of Physics, vol. 41, no. 6, pp. 65801, 2020. [Google Scholar]
2. S. Yang, R. Li, H. Li, K. Xu, Y. Shi et al., “Exploring the use of brain-computer interfaces in stroke neurorehabilitation,” BioMed Research International, vol. 2021, no. 6, pp. 1–11, 2021. [Google Scholar]
3. E. A. Katyal and R. Singla, “EEG-based hybrid QWERTY mental speller with high information transfer rate,” Medical & Biological Engineering & Computing, vol. 59, no. 3, pp. 633–661, 2021. [Google Scholar]
4. G. A. Worrell, B. Brinkmann and J. J. Van Gompel, “Investigational devices for epilepsy,” in Epilepsy Case Studies. Springer, Cham (Switzerlandpp. 217–221, 2021. [Google Scholar]
5. N. Mathur, A. Gupta, S. Jaswal and R. Verma, “Deep learning helps EEG signals predict different stages of visual processing in the human brain,” Biomedical Signal Processing and Control, vol. 70, no. 1, pp. 102996, 2021. [Google Scholar]
6. S. Park, J. Ha, H.-S. Cha, K.-G. Lee and C.-H. Im, “Development of an online home appliance control system for the elderly based on SSVEP-based brain-computer interface: A feasibility study,” in 2021 9th Int. Winter Conf. on Brain-Computer Interface (BCI), IEEE, Gangwon, Korea (Southpp. 1–2, 2021. [Google Scholar]
7. S. Mouli, R. Palaniappan, E. Molefi and I. McLoughlin, “In-ear electrode EEG for practical SSVEP BCI,” Technologies, vol. 8, no. 4, pp. 63, 2020. [Google Scholar]
8. A. H. Alsharif, N. Z. M. Salleh, R. Baharun, E. A.R.Hashem, A. A. Mansor et al., “Neuroimaging techniques in advertising research: main applications, development, and brain regions and processes,” Sustainability, vol. 13, no. 11, pp. 6488, 2021. [Google Scholar]
9. M. A. Lopez-Gordo, D. Sanchez-Morillo and F. P. Valle, “Dry EEG electrodes,” Sensors, vol. 14, no. 7, pp. 12847–12870, 2014. [Google Scholar]
10. S. Debener, F. Minow, R. Emkes, K. Gandras and M. De Vos, “How about taking a low-cost, small, and wireless EEG for a walk?,” Psychophysiology, vol. 49, no. 11, pp. 1617–1621, 2012. [Google Scholar]
11. P. Arico, G. Borghini, G. Di Flumeri, N. Sciaraffa, A. Colosimo et al., “Passive BCI in operational environments: insights, recent advances, and future trends,” IEEE Transactions on Biomedical Engineering, vol. 64, no. 7, pp. 1431–1436, 2017. [Google Scholar]
12. C. Ranganathan and S. Ganapathy, “Key dimensions of business-to-consumer web sites,” Information & Management, vol. 39, no. 6, pp. 457–465, 2002. [Google Scholar]
13. N. S. Suhaimi, J. Mountstephens and J. Teo, “EEG-based emotion recognition: A state-of-the-art review of current trends and opportunities,” Computational Intelligence and Neuroscience, vol. 2020, no. 6, pp. 1–19, 2020. [Google Scholar]
14. H. Abbaspour, N. Mehrshad, S. M. Razavi and L. Mesin, “Artefacts removal to detect visual evoked potentials in brain computer interface systems,” Journal of Biomimetics, Biomaterials and Biomedical Engineering, vol. 41, pp. 91–103, Trans Tech Publ, 2019. [Google Scholar]
15. S. Park, C.-H. Han and C.-H. Im, “Design of wearable EEG devices specialized for passive brain-computer interface applications,” Sensors, vol. 20, no. 16, pp. 4572, 2020. [Google Scholar]
16. S. Park, D.-W. Kim, C.-H. Han and C.-H. Im, “Estimation of emotional arousal changes of a group of individuals during movie screening using steady-state visual-evoked potential,” Frontiers in Neuroinformatics, vol. 15, pp. 44, 2021. [Google Scholar]
17. A. Y. Paek, J. A. Brantley, B. J. Evans and J. L. Contreras-Vidal, “Concerns in the blurred divisions between medical and consumer neurotechnology,” IEEE Systems Journal, vol. 15, no. 2, pp. 3069–3080, 2020. [Google Scholar]
18. G. A. M. Vasiljevic and L. C. de Miranda, “Brain-computer interface games based on consumer-grade EEG Devices: A systematic literature review,” International Journal of Human-Computer Interaction, vol. 36, no. 2, pp. 105–142, 2020. [Google Scholar]
19. Q. Lin, S. Song, I. D. Castro, H. Jiang, M. Konijnenburg et al., “Wearable multiple modality bio-signal recording and processing on chip: A review,” IEEE Sensors Journal, vol. 21, no. 2, pp. 1108–1123, 2020. [Google Scholar]
20. P. Sawangjai, S. Hompoonsup, P. Leelaarporn, S. Kongwudhikunakorn and T. Wilaiprasitporn, “Consumer grade EEG measuring sensors as research tools: A review,” IEEE Sensors Journal, vol. 20, no. 8, pp. 3996–4024, 2019. [Google Scholar]
21. M. Ogino and Y. Mitsukura, “Portable drowsiness detection through use of a prefrontal single-channel electroencephalogram,” Sensors, vol. 18, no. 12, pp. 4477, 2018. [Google Scholar]
22. P. Stapleton, J. Dispenza, S. McGill, D. Sabot, M. Peach et al., “Large effects of brief meditation intervention on EEG spectra in meditation novices,” IBRO Reports, vol. 9, no. 6, pp. 290–301, 2020. [Google Scholar]
23. J. W. Kam, S. Griffin, A. Shen, S. Patel, H. Hinrichs et al., “Systematic comparison between a wireless EEG system with dry electrodes and a wired EEG system with wet electrodes,” NeuroImage, vol. 184, pp. 119–129, 2019. [Google Scholar]
24. K. H. Khng and R. Mane, “Beyond BCI—Validating a wireless, consumer-grade EEG headset against a medical-grade system for evaluating EEG effects of a test anxiety intervention in school,” Advanced Engineering Informatics, vol. 45, no. Nov, pp. 101106, 2020. [Google Scholar]
25. J. Chen, D. Jiang, Y. Zhang and P. Zhang, “Emotion recognition from spatiotemporal EEG representations with hybrid convolutional recurrent neural networks via wearable multi-channel headset,” Computer Communications, vol. 154, no. 5, pp. 58–65, 2020. [Google Scholar]
26. T. Radüntz, “Signal quality evaluation of emerging EEG devices,” Frontiers in Physiology, vol. 9, pp. 98, 2018. [Google Scholar]
27. A. H. Alsharif, N. Z. M. Salleh and R. Baharun, “Neuromarketing: Marketing research in the new millennium,” Neuroscience Research Notes, vol. 4, no. 3, pp. 27–35, 2021. [Google Scholar]
28. A. H. Alsharif, N. Z. M. Salleh and R. Baharun, “Neuromarketing: The popularity of the brain-imaging and physiological tools,” Neuroscience Research Notes, vol. 3, no. 5, pp. 13–22, 2021. [Google Scholar]
29. A. Cruz, G. Pires, A. Lopes, C. Carona and U. J. Nunes, “A self-paced BCI with a collaborative controller for highly reliable wheelchair driving: Experimental tests with physically disabled individuals,” IEEE Transactions on Human-Machine Systems, vol. 51, no. 2, pp. 109–119, 2021. [Google Scholar]
30. M. K. Ghumman, S. Singh, N. Singh and B. Jindal, “Optimization of parameters for improving the performance of EEG-based BCI system,” Journal of Reliable Intelligent Environments, vol. 7, no. 2, pp. 145–156, 2021. [Google Scholar]
31. M. T. Sadiq, X. Yu, Z. Yuan and M. Z. Aziz, “Motor imagery BCI classification based on novel two-dimensional modelling in empirical wavelet transform,” Electronics Letters, vol. 56, no. 25, pp. 1367–1369, 2020. [Google Scholar]
32. D. Yadav, S. Yadav and K. Veer, “A comprehensive assessment of brain computer interfaces: Recent trends and challenges,” Journal of Neuroscience Methods, vol. 346, pp. 108918, 2020. [Google Scholar]
33. N. Veena and N. Anitha, “A review of non-invasive BCI devices,” International Journal Biomedical Engineering and Technology, vol. 34, no. 3, pp. 205, 2020. [Google Scholar]
34. A. Chamanzar, M. Behrmann and P. Grover, “Neural silences can be localized rapidly using noninvasive scalp EEG,” Communications Biology, vol. 4, no. 1, pp. 1–17, 2021. [Google Scholar]
35. M. T. Sadiq, X. Yu, Z. Yuan, Z. Fan, A. U. Rehman et al., “Motor imagery EEG signals classification based on mode amplitude and frequency components using empirical wavelet transform,” IEEE Access, vol. 7, pp. 127678–127692, 2019. [Google Scholar]
36. E. Butkevičiūtė, L. Bikulčienė, T. Sidekerskienė, T. Blažauskas, R. Maskeliūnas et al., “Removal of movement artefact for mobile EEG analysis in sports exercises,” IEEE Access, vol. 7, pp. 7206–7217, 2019. [Google Scholar]
37. X. Yu, M. Z. Aziz, M. T. Sadiq, Z. Fan and G. Xiao, “A new framework for automatic detection of motor and mental imagery EEG signals for robust BCI systems,” IEEE Transactions on Instrumentation and Measurement, vol. 70, pp. 1–12, 2021. [Google Scholar]
38. N. M. Krishna, K. Sekaran, A. V. N. Vamsi, G. P. Ghantasala, P. Chandana et al., “An efficient mixture model approach in brain-machine interface systems for extracting the psychological status of mentally impaired persons using EEG signals,” IEEE Access, vol. 7, pp. 77905–77914, 2019. [Google Scholar]
39. M. T. Sadiq, X. Yu and Z. Yuan, “Exploiting dimensionality reduction and neural network techniques for the development of expert brain-computer interfaces,” Expert Systems with Applications, vol. 164, no. 10, pp. 114031, 2021. [Google Scholar]
40. M. Seeck, L. Koessler, T. Bast, F. Leijten, C. Michel et al., “The standardized EEG electrode array of the IFCN,” Clinical Neurophysiology, vol. 128, no. 10, pp. 2070–2077, 2017. [Google Scholar]
41. R. Pasricha, S. Gupta and A. K. Srivastava, “A facile and novel synthesis of Ag-graphene-based nanocomposites,” Small, vol. 5, no. 20, pp. 2253–2259, 2009. [Google Scholar]
42. OpenBCI, OpenBCI Cyton Board, 2020. [Online]. Available: https://docs.openbci.com/Cyton/CytonLanding/. [Google Scholar]
43. D. Planchard, Engineering Design with SOLIDWORKS 2020. SDC Publications, Mission, Kansas, 2019. [Google Scholar]
44. C. H. Kellner, Handbook of ECT: A Guide to Electroconvulsive Therapy for Practitioners. Cambridge University Press, Cambridge, England, 2018. [Google Scholar]
45. N. Jamil, A. N. Belkacem, S. Ouhbi and A. Lakas, “Noninvasive electroencephalography equipment for assistive, adaptive, and rehabilitative brain-computer interfaces: A systematic literature review,” Sensors, vol. 21, no. 14, pp. 4754, 2021. [Google Scholar]
46. F. Nijboer, B. Van De Laar, S. Gerritsen, A. Nijholt and M. Poel, “Usability of three electroencephalogram headsets for brain-computer interfaces: A within subject comparison,” Interacting with Computers, vol. 27, no. 5, pp. 500–511, 2015. [Google Scholar]
47. M. T. Knierim, C. Berger and P. Reali, “Open-source concealed EEG data collection for brain-computer-interfaces--real-world neural observation through openBCI amplifiers with around-the-Ear cEEGrid electrodes,” arXiv preprint arXiv:2102.00414, 2021. [Google Scholar]
48. A. Vourvopoulos and S. B. I. Badia, “Usability and cost-effectiveness in brain-computer interaction: Is it user throughput or technology related?,” in Proc. of the 7th Augmented Human Int. Conf. 2016, Geneva, Switzerland, pp. 1–8, 2016. [Google Scholar]
49. M. T. Sadiq, X. Yu, Z. Yuan, M. Z. Aziz, S. Siuly et al., “Toward the development of versatile brain-computer interfaces,” IEEE Transactions on Artificial Intelligence, vol. 2, no. 4, pp. 314–328, 2021. [Google Scholar]
Cite This Article
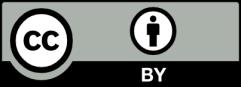