Open Access
ARTICLE
Robust Symmetry Prediction with Multi-Modal Feature Fusion for Partial Shapes
1 National University of Defense Technology, Changsha, Hunan, China
2 Jiangxi University of Finance and Economics, Jiangxi, China
3 Unit 78111 of Chinese People’s Liberation Army, Chengdu, Sichuan, China
4 Sungkyunkwan University, Korea
* Corresponding Author: Zhiping Cai. Email:
Intelligent Automation & Soft Computing 2023, 35(3), 3099-3111. https://doi.org/10.32604/iasc.2023.030298
Received 23 March 2022; Accepted 28 April 2022; Issue published 17 August 2022
Abstract
In geometry processing, symmetry research benefits from global geometric features of complete shapes, but the shape of an object captured in real-world applications is often incomplete due to the limited sensor resolution, single viewpoint, and occlusion. Different from the existing works predicting symmetry from the complete shape, we propose a learning approach for symmetry prediction based on a single RGB-D image. Instead of directly predicting the symmetry from incomplete shapes, our method consists of two modules, i.e., the multi-modal feature fusion module and the detection-by-reconstruction module. Firstly, we build a channel-transformer network (CTN) to extract cross-fusion features from the RGB-D as the multi-modal feature fusion module, which helps us aggregate features from the color and the depth separately. Then, our self-reconstruction network based on a 3D variational auto-encoder (3D-VAE) takes the global geometric features as input, followed by a prediction symmetry network to detect the symmetry. Our experiments are conducted on three public datasets: ShapeNet, YCB, and ScanNet, we demonstrate that our method can produce reliable and accurate results.Keywords
Cite This Article
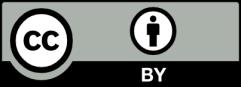