Open Access
ARTICLE
Anomaly Detection in Social Media Texts Using Optimal Convolutional Neural Network
1 Department of Computer Science and Engineering, Ramco Institute of Technology, Rajapalayam, 626117, India
2 Department of Electronics and Communication Engineering, P. S. R. Engineering College, Sivakasi, 626140, India
* Corresponding Author: Valarmathi Krishnasamy. Email:
Intelligent Automation & Soft Computing 2023, 36(1), 1027-1042. https://doi.org/10.32604/iasc.2023.031165
Received 11 April 2022; Accepted 05 July 2022; Issue published 29 September 2022
Abstract
Social Networking Sites (SNSs) are nowadays utilized by the whole world to share ideas, images, and valuable contents by means of a post to reach a group of users. The use of SNS often inflicts the physical and the mental health of the people. Nowadays, researchers often focus on identifying the illegal behaviors in the SNS to reduce its negative influence. The state-of-art Natural Language processing techniques for anomaly detection have utilized a wide annotated corpus to identify the anomalies and they are often time-consuming as well as certainly do not guarantee maximum accuracy. To overcome these issues, the proposed methodology utilizes a Modified Convolutional Neural Network (MCNN) using stochastic pooling and a Leaky Rectified Linear Unit (LReLU). Here, each word in the social media text is analyzed based on its meaning. The stochastic pooling accurately detects the anomalous social media posts and reduces the chance of overfitting. The LReLU overcomes the high computational cost and gradient vanishing problem associated with other activation functions. It also doesn’t stop the learning process when the values are negative. The MCNN computes a specified score value using a novel integrated anomaly detection technique. Based on the score value, the anomalies are identified. A Teaching Learning based Optimization (TLBO) algorithm has been used to optimize the feature extraction phase of the modified CNN and fast convergence is offered. In this way, the performance of the model is enhanced in terms of classification accuracy. The efficiency of the proposed technique is compared with the state-of-art techniques in terms of accuracy, sensitivity, specificity, recall, and precision. The proposed MCNN-TLBO technique has provided an overall architecture of 97.85%, 95.45%, and 97.55% for the three social media datasets namely Facebook, Twitter, and Reddit respectively.Keywords
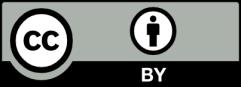