Open Access
ARTICLE
Hybrid Convolutional Neural Network for Plant Diseases Prediction
1 Department of Information Technology, Sri Sivasubramaniya Nadar College of Engineering, Chennai, 603110, India
2 Department of Statistics and Operations Research, College of Science, King Saud University, Riyadh, 11451, Saudi Arabia
3 Department of Mathematics, Faculty of Science, Mansoura University, Mansoura, 35516, Egypt
4 Department of Computational Mathematics, Science, and Engineering (CMSE), Michigan State University, East Lansing, MI, 48824, USA
* Corresponding Author: S. Poornima. Email:
Intelligent Automation & Soft Computing 2023, 36(2), 2393-2409. https://doi.org/10.32604/iasc.2023.024820
Received 01 November 2021; Accepted 11 January 2022; Issue published 05 January 2023
Abstract
Plant diseases prediction is the essential technique to prevent the yield loss and gain high production of agricultural products. The monitoring of plant health continuously and detecting the diseases is a significant for sustainable agriculture. Manual system to monitor the diseases in plant is time consuming and report a lot of errors. There is high demand for technology to detect the plant diseases automatically. Recently image processing approach and deep learning approach are highly invited in detection of plant diseases. The diseases like late blight, bacterial spots, spots on Septoria leaf and yellow leaf curved are widely found in plants. These are the main reasons to affects the plants life and yield. To identify the diseases earliest, our research presents the hybrid method by combining the region based convolutional neural network (RCNN) and region based fully convolutional networks (RFCN) for classifying the diseases. First the leaf images of plants are collected and preprocessed to remove noisy data in image. Further data normalization, augmentation and removal of background noises are done. The images are divided as testing and training, training images are fed as input to deep learning architecture. First, we identify the region of interest (RoI) by using selective search. In every region, feature of convolutional neural network (CNN) is extracted independently for further classification. The plants such as tomato, potato and bell pepper are taken for this experiment. The plant input image is analyzed and classify as healthy plant or unhealthy plant. If the image is detected as unhealthy, then type of diseases the plant is affected will be displayed. Our proposed technique achieves 98.5% of accuracy in predicting the plant diseases.Keywords
Cite This Article
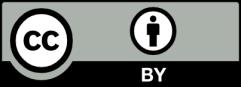