Open Access
ARTICLE
Word Sense Disambiguation Based Sentiment Classification Using Linear Kernel Learning Scheme
1 Anna University, Chennai, 600025, Tamil Nadu, India
2 Sona College of Technology, Salem, 636005, Tamilnadu, India
* Corresponding Author: P. Ramya. Email:
Intelligent Automation & Soft Computing 2023, 36(2), 2379-2391. https://doi.org/10.32604/iasc.2023.026291
Received 21 December 2021; Accepted 24 February 2022; Issue published 05 January 2023
Abstract
Word Sense Disambiguation has been a trending topic of research in Natural Language Processing and Machine Learning. Mining core features and performing the text classification still exist as a challenging task. Here the features of the context such as neighboring words like adjective provide the evidence for classification using machine learning approach. This paper presented the text document classification that has wide applications in information retrieval, which uses movie review datasets. Here the document indexing based on controlled vocabulary, adjective, word sense disambiguation, generating hierarchical categorization of web pages, spam detection, topic labeling, web search, document summarization, etc. Here the kernel support vector machine learning algorithm helps to classify the text and feature extract is performed by cuckoo search optimization. Positive review and negative review of movie dataset is presented to get the better classification accuracy. Experimental results focused with context mining, feature analysis and classification. By comparing with the previous work, proposed work designed to achieve the efficient results. Overall design is performed with MATLAB 2020a tool.Keywords
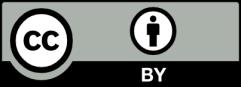