Open Access
ARTICLE
Deep Capsule Residual Networks for Better Diagnosis Rate in Medical Noisy Images
1 Department of Electronics and Communication Engineering, Anna University, Chennai, 600025, India
2 Department of Electronics and Communication Engineering, M.Kumarasamy College of Engineering, Karur, 639113, India
* Corresponding Author: P. S. Arthy. Email:
Intelligent Automation & Soft Computing 2023, 36(3), 2959-2971. https://doi.org/10.32604/iasc.2023.032511
Received 20 May 2022; Accepted 04 August 2022; Issue published 15 March 2023
Abstract
With the advent of Machine and Deep Learning algorithms, medical image diagnosis has a new perception of diagnosis and clinical treatment. Regrettably, medical images are more susceptible to capturing noises despite the peak in intelligent imaging techniques. However, the presence of noise images degrades both the diagnosis and clinical treatment processes. The existing intelligent methods suffer from the deficiency in handling the diverse range of noise in the versatile medical images. This paper proposes a novel deep learning network which learns from the substantial extent of noise in medical data samples to alleviate this challenge. The proposed deep learning architecture exploits the advantages of the capsule network, which is used to extract correlation features and combine them with redefined residual features. Additionally, the final stage of dense learning is replaced with powerful extreme learning machines to achieve a better diagnosis rate, even for noisy and complex images. Extensive experimentation has been conducted using different medical images. Various performances such as Peak-Signal-To-Noise Ratio (PSNR) and Structural-Similarity-Index-Metrics (SSIM) are compared with the existing deep learning architectures. Additionally, a comprehensive analysis of individual algorithms is analyzed. The experimental results prove that the proposed model has outperformed the other existing algorithms by a substantial margin and proved its supremacy over the other learning models.Keywords
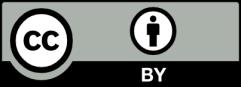