Open Access
ARTICLE
Automatic Team Assignment and Jersey Number Recognition in Football Videos
1 Faculty of Computing and Information Technology, University of Tabuk, Tabuk, 47321, Saudi Arabia
2 Industrial Innovation & Robotics Center (IIRC), University of Tabuk, Saudi Arabia
* Corresponding Author: Saleh Albelwi. Email:
Intelligent Automation & Soft Computing 2023, 36(3), 2669-2684. https://doi.org/10.32604/iasc.2023.033062
Received 06 June 2022; Accepted 14 November 2022; Issue published 15 March 2023
Abstract
Football is one of the most-watched sports, but analyzing players’ performance is currently difficult and labor intensive. Performance analysis is done manually, which means that someone must watch video recordings and then log each player’s performance. This includes the number of passes and shots taken by each player, the location of the action, and whether or not the play had a successful outcome. Due to the time-consuming nature of manual analyses, interest in automatic analysis tools is high despite the many interdependent phases involved, such as pitch segmentation, player and ball detection, assigning players to their teams, identifying individual players, activity recognition, etc. This paper proposes a system for developing an automatic video analysis tool for sports. The proposed system is the first to integrate multiple phases, such as segmenting the field, detecting the players and the ball, assigning players to their teams, and identifying players’ jersey numbers. In team assignment, this research employed unsupervised learning based on convolutional autoencoders (CAEs) to learn discriminative latent representations and minimize the latent embedding distance between the players on the same team while simultaneously maximizing the distance between those on opposing teams. This paper also created a highly accurate approach for the real-time detection of the ball. Furthermore, it also addressed the lack of jersey number datasets by creating a new dataset with more than 6,500 images for numbers ranging from 0 to 99. Since achieving a high performance in deep learning requires a large training set, and the collected dataset was not enough, this research utilized transfer learning (TL) to first pretrain the jersey number detection model on another large dataset and then fine-tune it on the target dataset to increase the accuracy. To test the proposed system, this paper presents a comprehensive evaluation of its individual stages as well as of the system as a whole. All codes, datasets, and experiments are available on GitHub ().Keywords
Cite This Article
R. Alhejaily, R. Alhejaily, M. Almdahrsh, S. Alessa and S. Albelwi, "Automatic team assignment and jersey number recognition in football videos," Intelligent Automation & Soft Computing, vol. 36, no.3, pp. 2669–2684, 2023. https://doi.org/10.32604/iasc.2023.033062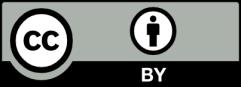