Open Access
ARTICLE
A Novel Deep Learning Representation for Industrial Control System Data
1 Key Laboratory of Networked Control Systems, Chinese Academy of Sciences, Shenyang, 110016, China
2 Shenyang Institute of Automation, Chinese Academy of Sciences, Shenyang, 110016, China
3 Institutes for Robotics and Intelligent Manufacturing, Chinese Academy of Sciences, Shenyang, 110169, China
4 Shenyang Aircraft Corporation, Shenyang, 110850, China
5 Molarray Research, Toronto, L4B3K1, Canada
* Corresponding Author: Jianming Zhao. Email:
Intelligent Automation & Soft Computing 2023, 36(3), 2703-2717. https://doi.org/10.32604/iasc.2023.033762
Received 27 June 2022; Accepted 11 November 2022; Issue published 15 March 2023
Abstract
Feature extraction plays an important role in constructing artificial intelligence (AI) models of industrial control systems (ICSs). Three challenges in this field are learning effective representation from high-dimensional features, data heterogeneity, and data noise due to the diversity of data dimensions, formats and noise of sensors, controllers and actuators. Hence, a novel unsupervised learning autoencoder model is proposed for ICS data in this paper. Although traditional methods only capture the linear correlations of ICS features, our deep industrial representation learning model (DIRL) based on a convolutional neural network can mine high-order features, thus solving the problem of high-dimensional and heterogeneous ICS data. In addition, an unsupervised denoising autoencoder is introduced for noisy ICS data in DIRL. Training the denoising autoencoder allows the model to better mitigate the sensor noise problem. In this way, the representative features learned by DIRL could help to evaluate the safety state of ICSs more effectively. We tested our model with absolute and relative accuracy experiments on two large-scale ICS datasets. Compared with other popular methods, DIRL showed advantages in four common indicators of AI algorithms: accuracy, precision, recall, and F1-score. This study contributes to the effective analysis of large-scale ICS data, which promotes the stable operation of ICSs.Keywords
Cite This Article
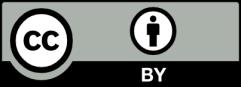