Open Access
ARTICLE
Secured Framework for Assessment of Chronic Kidney Disease in Diabetic Patients
Dean of the College of Arts and Sciences, Prince Sattam Bin Abdulaziz University, Wadi Al-Dawasir, 11990, Saudi Arabia
* Corresponding Author: Sultan Mesfer Aldossary. Email:
Intelligent Automation & Soft Computing 2023, 36(3), 3387-3404. https://doi.org/10.32604/iasc.2023.035249
Received 13 August 2022; Accepted 04 November 2022; Issue published 15 March 2023
Abstract
With the emergence of cloud technologies, the services of healthcare systems have grown. Simultaneously, machine learning systems have become important tools for developing matured and decision-making computer applications. Both cloud computing and machine learning technologies have contributed significantly to the success of healthcare services. However, in some areas, these technologies are needed to provide and decide the next course of action for patients suffering from diabetic kidney disease (DKD) while ensuring privacy preservation of the medical data. To address the cloud data privacy problem, we proposed a DKD prediction module in a framework using cloud computing services and a data control scheme. This framework can provide improved and early treatment before end-stage renal failure. For prediction purposes, we implemented the following machine learning algorithms: support vector machine (SVM), random forest (RF), decision tree (DT), naïve Bayes (NB), deep learning (DL), and k nearest neighbor (KNN). These classification techniques combined with the cloud computing services significantly improved the decision making in the progress of DKD patients. We applied these classifiers to the UCI Machine Learning Repository for chronic kidney disease using various clinical features, which are categorized as single, combination of selected features, and all features. During single clinical feature experiments, machine learning classifiers SVM, RF, and KNN outperformed the remaining classification techniques, whereas in combined clinical feature experiments, the maximum accuracy was achieved for the combination of DL and RF. All the feature experiments presented increased accuracy and increased F-measure metrics from SVM, DL, and RF.Keywords
Cite This Article
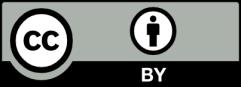