Open Access
ARTICLE
Feature Fusion Based Deep Transfer Learning Based Human Gait Classification Model
1 Department of Electronics and Instrumentation Engineering, V. R. Siddhartha Engineering College, Vijayawada, 520007, India
2 Candidate of Pedagogical Sciences, Department of Industrial Electronics and Lighting Engineering, Kazan State Power Engineering University, Kazan, 420066, Russia
3 Department of Theories and Principles of Physical Education and Life Safety, North-Eastern Federal University Named After M. K. Ammosov, Yakutsk, 677000, Russia
4 Department of Computer Science and Engineering, Vignan’s Institute of Information Technology, Visakhapatnam, 530049, India
5 Department of Applied Data Science, Noroff University College, Kristiansand, Norway
6 Artificial Intelligence Research Center (AIRC), Ajman University, Ajman, 346, United Arab Emirates
7 Department of Electrical and Computer Engineering, Lebanese American University, Byblos, Lebanon
8 Department of ICT Convergence, Soonchunhyang University, Asan, 31538, Korea
* Corresponding Author: Yunyoung Nam. Email:
Intelligent Automation & Soft Computing 2023, 37(2), 1453-1468. https://doi.org/10.32604/iasc.2023.038321
Received 07 December 2022; Accepted 16 January 2023; Issue published 21 June 2023
Abstract
Gait is a biological typical that defines the method by that people walk. Walking is the most significant performance which keeps our day-to-day life and physical condition. Surface electromyography (sEMG) is a weak bioelectric signal that portrays the functional state between the human muscles and nervous system to any extent. Gait classifiers dependent upon sEMG signals are extremely utilized in analysing muscle diseases and as a guide path for recovery treatment. Several approaches are established in the works for gait recognition utilizing conventional and deep learning (DL) approaches. This study designs an Enhanced Artificial Algae Algorithm with Hybrid Deep Learning based Human Gait Classification (EAAA-HDLGR) technique on sEMG signals. The EAAA-HDLGR technique extracts the time domain (TD) and frequency domain (FD) features from the sEMG signals and is fused. In addition, the EAAA-HDLGR technique exploits the hybrid deep learning (HDL) model for gait recognition. At last, an EAAA-based hyperparameter optimizer is applied for the HDL model, which is mainly derived from the quasi-oppositional based learning (QOBL) concept, showing the novelty of the work. A brief classifier outcome of the EAAA-HDLGR technique is examined under diverse aspects, and the results indicate improving the EAAA-HDLGR technique. The results imply that the EAAA-HDLGR technique accomplishes improved results with the inclusion of EAAA on gait recognition.Keywords
Cite This Article
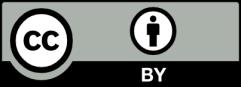